有关twin的svr的Matlab
时间: 2024-04-03 08:31:53 浏览: 23
您好!关于 twin SVR 的 Matlab,我可以给您一些简单的介绍。
首先,twin SVR 是一种支持向量回归(SVR)的变体,它可以在训练过程中同时考虑正例和负例的误差。相比于传统的 SVR,twin SVR 能够更好地处理正例和负例在数据集中分布不均的情况。
在 Matlab 中,您可以使用相关的工具箱(如 Statistics and Machine Learning Toolbox 或者 Support Package for Azure)来实现 twin SVR。具体地,您可以按照以下步骤进行操作:
1. 准备数据集:从数据集中加载训练数据和测试数据,并将它们分别存储为矩阵或表格的形式。
2. 设置模型参数:设置 twin SVR 模型的参数,如正则化参数、核函数等。这些参数可以通过交叉验证等方法来调整。
3. 训练模型:使用训练数据集来训练 twin SVR 模型,并得到模型的系数和截距等参数。
4. 测试模型:使用测试数据集来测试训练好的 twin SVR 模型,并计算模型的预测误差和准确率等指标。
5. 优化模型:根据测试结果对模型进行优化,如调整模型参数、增加训练数据等。
以上是简单的步骤介绍,具体的实现细节还需要根据您的具体需求来进行调整和优化。希望这些信息能够对您有所帮助!
相关问题
svr matlab
SVR是支持向量机回归的缩写,是一种常用的机器学习算法。在MATLAB中,可以使用自带的SVR工具箱来实现SVR回归预测。具体流程包括以下几步:
1. 准备数据集,包括训练集和测试集。
2. 对数据进行归一化处理,可以使用MATLAB中的mapminmax函数。
3. 设置SVR的基本参数,包括惩罚系数c和核函数宽度g等。
4. 使用SVR工具箱进行训练和预测,可以使用MATLAB中的svrtrain和svmpredict函数。
需要注意的是,在使用SVR进行回归预测时,需要根据具体问题选择合适的核函数和参数,以达到最好的预测效果。
SVRmatlab程序
SVR(Support Vector Regression)是一种机器学习算法,用于回归问题。它基于支持向量机(SVM)算法,通过寻找一个最优的超平面来拟合数据,从而进行预测。
在Matlab中,可以使用libsvm库来实现SVR算法。libsvm是一个开源的机器学习库,提供了一系列用于支持向量机函数和工具。
下面是一个简单的SVR Matlab程序示例:
```matlab
% 导入libsvm库
addpath('libsvm');
% 加载训练数据
load('train_data.mat');
X_train = train_data(:, 1:end-1);
y_train = train_data(:, end);
% 加载测试数据
load('test_data.mat');
X_test = test_data(:, 1:end-1);
y_test = test_data(:, end);
% 训练SVR模型
model = svmtrain(y_train, X_train, '-s 3 -t 2');
% 预测
[y_pred, ~, ~] = svmpredict(y_test, X_test, model);
% 计算均方误差(MSE)
mse = mean((y_pred - y_test).^2);
% 显示结果
disp(['均方误差:', num2str(mse)]);
% 清除libsvm库路径
rmpath('libsvm');
```
在上面的示例中,首先需要导入libsvm库,并加载训练数据和测试数据。然后使用`svmtrain`函数训练SVR模型,其中`'-s 3 -t 2'`是训练参数,表示使用epsilon-SVR模型和RBF核函数。接下来使用`svmpredict`函数进行预测,并计算均方误差(MSE)。最后显示结果并清除libsvm库路径。
希望以上示例对你有所帮助!
相关推荐
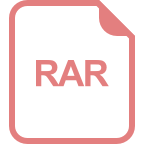
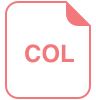
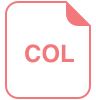
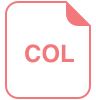
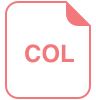
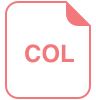







