bmp图片压缩matlab
时间: 2023-11-26 09:48:04 浏览: 31
根据提供的引用内容,bmp图片压缩的Matlab实现可以使用BP神经网络和DCT算法。具体步骤如下:
1.使用Matlab读取bmp图片,并将其转换为灰度图像。
2.将灰度图像的像素值减去128,以便于后续处理。
3.将图像分成8x8的块,对每个块进行DCT变换。
4.对DCT系数进行量化,可以使用标准的JPEG量化矩阵。
5.使用BP神经网络对量化后的DCT系数进行编码。
6.将编码后的数据写入文件中。
下面是一个简单的Matlab代码示例,用于对bmp图片进行压缩:
```matlab
% 读取bmp图片
img = imread('lena.bmp');
% 转换为灰度图像
gray_img = rgb2gray(img);
% 减去128
gray_img = gray_img - 128;
% 分成8x8的块,对每个块进行DCT变换
dct_img = blkproc(gray_img, [8 8], @dct2);
% 量化DCT系数
quantization_matrix = [16 11 10 16 24 40 51 61;
12 12 14 19 26 58 60 55;
14 13 16 24 40 57 69 56;
14 17 22 29 51 87 80 62;
18 22 37 56 68 109 103 77;
24 35 55 64 81 104 113 92;
49 64 78 87 103 121 120 101;
72 92 95 98 112 100 103 99];
quantized_dct_img = blkproc(dct_img, [8 8], @(block_struct) round(block_struct.data ./ quantization_matrix));
% 使用BP神经网络对量化后的DCT系数进行编码
net = newff(minmax(quantized_dct_img(:)'), [20 1], {'logsig', 'purelin'}, 'trainlm');
net.trainParam.show = 50;
net.trainParam.lr = 0.05;
net.trainParam.epochs = 500;
net.trainParam.goal = 0.001;
net = train(net, quantized_dct_img(:)', quantized_dct_img(:)');
% 将编码后的数据写入文件中
encoded_data = sim(net, quantized_dct_img(:)');
fid = fopen('compressed_data.bin', 'wb');
fwrite(fid, encoded_data, 'double');
fclose(fid);
```
相关推荐
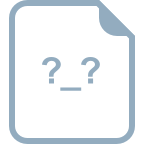
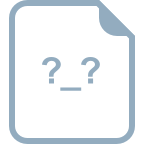
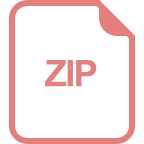














