代码讲解 def print_results(dataset, y_pred, matches, proba): results = [] for i in range(dataset.shape[0]): print() if y_pred[i] == 2: print(matches.iloc[i, 0] + " vs. " + matches.iloc[i, 1] + " => Draw") results.append({'result': 'Draw'}) elif y_pred[i] == 1: print(matches.iloc[i, 0] + " vs. " + matches.iloc[i, 1] + " => Winner: " + dataset.iloc[i, 0]) results.append({'result': dataset.iloc[i, 0]}) else: print(matches.iloc[i, 0] + " vs. " + matches.iloc[i, 1] + " => Winner: " + dataset.iloc[i, 1]) results.append({'result': dataset.iloc[i, 1]}) try: print('Probability of ' + dataset.iloc[i, 0] + ' winning: ', '%.3f'%(proba[i][1])) print('Probability of Draw: ', '%.3f'%(proba[i][2])) print('Probability of ' + dataset.iloc[i, 1] + ' winning: ', '%.3f'%(proba[i][0])) except: print('Probability of ' + dataset.iloc[i, 1] + ' winning: ', '%.3f'%(proba[i][0])) print("") results = pd.DataFrame(results) matches = pd.concat([matches.group, results], axis=1) return matches
时间: 2023-07-14 11:14:06 浏览: 45
这个函数的主要作用是打印出预测结果,并将结果存储在一个 Pandas DataFrame 中返回。
输入参数包括一个数据集 dataset,一个数组 y_pred 表示模型对数据集进行的预测,一个 Pandas DataFrame matches 存储比赛对阵信息,以及一个数组 proba 表示模型预测每个结果的概率。
函数中的 for 循环遍历数据集中的每一行,根据预测结果打印出比赛胜负情况和概率,同时将比赛结果存储在一个列表中。
最后,将比赛结果列表转换成一个 Pandas DataFrame,将其与比赛对阵信息合并,返回结果。
相关问题
帮我为下面的代码加上注释:class SimpleDeepForest: def __init__(self, n_layers): self.n_layers = n_layers self.forest_layers = [] def fit(self, X, y): X_train = X for _ in range(self.n_layers): clf = RandomForestClassifier() clf.fit(X_train, y) self.forest_layers.append(clf) X_train = np.concatenate((X_train, clf.predict_proba(X_train)), axis=1) return self def predict(self, X): X_test = X for i in range(self.n_layers): X_test = np.concatenate((X_test, self.forest_layers[i].predict_proba(X_test)), axis=1) return self.forest_layers[-1].predict(X_test[:, :-2]) # 1. 提取序列特征(如:GC-content、序列长度等) def extract_features(fasta_file): features = [] for record in SeqIO.parse(fasta_file, "fasta"): seq = record.seq gc_content = (seq.count("G") + seq.count("C")) / len(seq) seq_len = len(seq) features.append([gc_content, seq_len]) return np.array(features) # 2. 读取相互作用数据并创建数据集 def create_dataset(rna_features, protein_features, label_file): labels = pd.read_csv(label_file, index_col=0) X = [] y = [] for i in range(labels.shape[0]): for j in range(labels.shape[1]): X.append(np.concatenate([rna_features[i], protein_features[j]])) y.append(labels.iloc[i, j]) return np.array(X), np.array(y) # 3. 调用SimpleDeepForest分类器 def optimize_deepforest(X, y): X_train, X_test, y_train, y_test = train_test_split(X, y, test_size=0.2) model = SimpleDeepForest(n_layers=3) model.fit(X_train, y_train) y_pred = model.predict(X_test) print(classification_report(y_test, y_pred)) # 4. 主函数 def main(): rna_fasta = "RNA.fasta" protein_fasta = "pro.fasta" label_file = "label.csv" rna_features = extract_features(rna_fasta) protein_features = extract_features(protein_fasta) X, y = create_dataset(rna_features, protein_features, label_file) optimize_deepforest(X, y) if __name__ == "__main__": main()
# Define a class named 'SimpleDeepForest'
class SimpleDeepForest:
# Initialize the class with 'n_layers' parameter
def __init__(self, n_layers):
self.n_layers = n_layers
self.forest_layers = []
# Define a method named 'fit' to fit the dataset into the classifier
def fit(self, X, y):
X_train = X
# Use the forest classifier to fit the dataset for 'n_layers' times
for _ in range(self.n_layers):
clf = RandomForestClassifier()
clf.fit(X_train, y)
# Append the classifier to the list of forest layers
self.forest_layers.append(clf)
# Concatenate the training data with the predicted probability of the last layer
X_train = np.concatenate((X_train, clf.predict_proba(X_train)), axis=1)
# Return the classifier
return self
# Define a method named 'predict' to make predictions on the test set
def predict(self, X):
X_test = X
# Concatenate the test data with the predicted probability of each layer
for i in range(self.n_layers):
X_test = np.concatenate((X_test, self.forest_layers[i].predict_proba(X_test)), axis=1)
# Return the predictions of the last layer
return self.forest_layers[-1].predict(X_test[:, :-2])
# Define a function named 'extract_features' to extract sequence features
def extract_features(fasta_file):
features = []
# Parse the fasta file to extract sequence features
for record in SeqIO.parse(fasta_file, "fasta"):
seq = record.seq
gc_content = (seq.count("G") + seq.count("C")) / len(seq)
seq_len = len(seq)
features.append([gc_content, seq_len])
# Return the array of features
return np.array(features)
# Define a function named 'create_dataset' to create the dataset
def create_dataset(rna_features, protein_features, label_file):
labels = pd.read_csv(label_file, index_col=0)
X = []
y = []
# Create the dataset by concatenating the RNA and protein features
for i in range(labels.shape[0]):
for j in range(labels.shape[1]):
X.append(np.concatenate([rna_features[i], protein_features[j]]))
y.append(labels.iloc[i, j])
# Return the array of features and the array of labels
return np.array(X), np.array(y)
# Define a function named 'optimize_deepforest' to optimize the deep forest classifier
def optimize_deepforest(X, y):
# Split the dataset into training set and testing set
X_train, X_test, y_train, y_test = train_test_split(X, y, test_size=0.2)
# Create an instance of the SimpleDeepForest classifier with 3 layers
model = SimpleDeepForest(n_layers=3)
# Fit the training set into the classifier
model.fit(X_train, y_train)
# Make predictions on the testing set
y_pred = model.predict(X_test)
# Print the classification report
print(classification_report(y_test, y_pred))
# Define the main function to run the program
def main():
rna_fasta = "RNA.fasta"
protein_fasta = "pro.fasta"
label_file = "label.csv"
# Extract the RNA and protein features
rna_features = extract_features(rna_fasta)
protein_features = extract_features(protein_fasta)
# Create the dataset
X, y = create_dataset(rna_features, protein_features, label_file)
# Optimize the DeepForest classifier
optimize_deepforest(X, y)
# Check if the program is being run as the main program
if __name__ == "__main__":
main()
能否优化以下程序import pandas as pd from sklearn.model_selection import train_test_split from sklearn.feature_extraction.text import CountVectorizer from sklearn.naive_bayes import MultinomialNB # 读取数据集 df = pd.read_csv('news_dataset.csv', error_bad_lines=False) # 划分数据集 X_train, X_test, y_train, y_test = train_test_split(df['text'], df['label'], test_size=0.2) # 将文本转换为数字向量 vectorizer = CountVectorizer() X_train_vec = vectorizer.fit_transform(X_train) X_test_vec = vectorizer.transform(X_test) # 训练朴素贝叶斯分类器 classifier = MultinomialNB() classifier.fit(X_train_vec, y_train) # 预测测试集 y_pred = classifier.predict(X_test_vec) # 计算准确率 accuracy = (y_pred == y_test).sum() / y_test.shape[0] print(f'Accuracy: {accuracy}')
这段代码已经很简洁了,但是还有一些可以优化的地方:
1. 使用Pandas的`read_csv`函数时,可以指定`dtype`参数,这样可以避免Pandas自动推断数据类型所需的时间。
2. 在划分数据集时,可以指定`random_state`参数,这样可以保证每次运行时数据集的划分是一样的。
3. 在将文本转换为数字向量时,可以使用`TfidfVectorizer`代替`CountVectorizer`,因为`TfidfVectorizer`可以考虑到词频和文档频率的影响。
4. 在预测测试集时,可以使用`predict_proba`函数获得每个样本属于各个类别的概率值,这样可以更加细致地评估模型的性能。
下面是优化后的代码:
```python
import pandas as pd
from sklearn.model_selection import train_test_split
from sklearn.feature_extraction.text import TfidfVectorizer
from sklearn.naive_bayes import MultinomialNB
# 读取数据集,指定数据类型
df = pd.read_csv('news_dataset.csv', error_bad_lines=False, dtype={'text': str, 'label': int})
# 划分数据集,指定随机种子
X_train, X_test, y_train, y_test = train_test_split(df['text'], df['label'], test_size=0.2, random_state=42)
# 将文本转换为数字向量,使用TfidfVectorizer
vectorizer = TfidfVectorizer()
X_train_vec = vectorizer.fit_transform(X_train)
X_test_vec = vectorizer.transform(X_test)
# 训练朴素贝叶斯分类器
classifier = MultinomialNB()
classifier.fit(X_train_vec, y_train)
# 预测测试集,使用predict_proba函数获取概率值
y_pred_prob = classifier.predict_proba(X_test_vec)
y_pred = y_pred_prob.argmax(axis=1)
# 计算准确率
accuracy = (y_pred == y_test).sum() / y_test.shape[0]
print(f'Accuracy: {accuracy}')
```
相关推荐
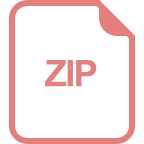







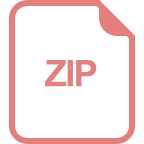
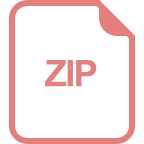
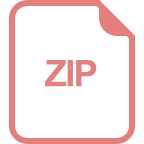
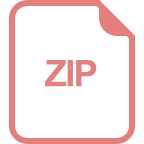
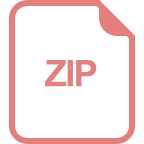