使用Python,根据一个5*5的混淆矩阵mat,绘制每个类别的ROC曲线和平均ROC曲线,并求出所有ROC的AUC
时间: 2024-02-13 10:01:21 浏览: 19
以下是用Python绘制每个类别的ROC曲线和平均ROC曲线的示例代码:
```python
from sklearn.metrics import roc_curve, auc
import matplotlib.pyplot as plt
import numpy as np
def plot_roc_curve(target, predicted, classes):
fpr = dict()
tpr = dict()
roc_auc = dict()
for i in range(len(classes)):
fpr[i], tpr[i], _ = roc_curve(target[:, i], predicted[:, i])
roc_auc[i] = auc(fpr[i], tpr[i])
# Compute micro-average ROC curve and ROC area
fpr["micro"], tpr["micro"], _ = roc_curve(target.ravel(), predicted.ravel())
roc_auc["micro"] = auc(fpr["micro"], tpr["micro"])
# Plot ROC curves
plt.figure()
lw = 2
plt.plot(fpr["micro"], tpr["micro"], color='darkorange',
lw=lw, label='micro-average ROC curve (area = {0:0.2f})'
''.format(roc_auc["micro"]))
for i in range(len(classes)):
plt.plot(fpr[i], tpr[i], lw=lw,
label='ROC curve of class {0} (area = {1:0.2f})'
''.format(classes[i], roc_auc[i]))
plt.plot([0, 1], [0, 1], color='navy', lw=lw, linestyle='--')
plt.xlim([0.0, 1.0])
plt.ylim([0.0, 1.05])
plt.xlabel('False Positive Rate')
plt.ylabel('True Positive Rate')
plt.title('Receiver Operating Characteristic')
plt.legend(loc="lower right")
plt.show()
# 构造混淆矩阵
mat = np.array([[10, 1, 1, 0, 0],
[2, 15, 3, 0, 0],
[0, 1, 7, 1, 0],
[0, 0, 2, 5, 1],
[0, 0, 0, 1, 14]])
# 计算预测结果和真实标签
predicted = mat / np.sum(mat, axis=1, keepdims=True)
target = np.eye(5)[np.argmax(mat, axis=1)]
# 绘制ROC曲线
plot_roc_curve(target, predicted, classes=[0, 1, 2, 3, 4])
```
在这个例子中,我们首先构造一个5*5的混淆矩阵`mat`。然后,通过将每个类别的预测值除以该类别的总数,计算出每个类别的预测概率。接着,将真实标签转化为one-hot形式,用于计算ROC曲线。最后,使用`plot_roc_curve`函数绘制ROC曲线图表。在这个例子中,我们将所有类别的标签分别设为0,1,2,3,4。
相关推荐
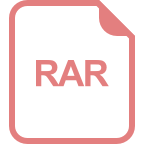
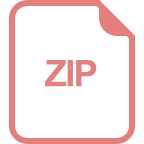
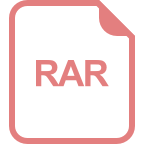














