When we use kmeans for image segmentation, the color information of pixels is used for clustering, so each of our pixels can be regarded as a vector composed of R, G, and B, and RGB is our color feature. The specific process is similar to our example above, but the calculation object is changed from a scalar to a 3-dimensional vector. Please implement the `kmean_color` function , ### Clustering Methods for colorful image def kmeans_color(features, k, num_iters=500): N=None # 像素个数 assignments = np.zeros(N, dtype=np.uint32) #Like the kmeans function above ### YOUR CODE HERE pass ### END YOUR CODE return assignments
时间: 2024-02-07 07:03:21 浏览: 27
Sure, here is a possible implementation of the `kmeans_color` function for image segmentation using the RGB color information:
```python
import numpy as np
def kmeans_color(features, k, num_iters=500):
# Get the number of pixels in the image
N = features.shape[0]
# Initialize the cluster centers randomly
centers = features[np.random.choice(N, k, replace=False)]
# Iterate until convergence or maximum number of iterations reached
for t in range(num_iters):
# Assign each pixel to the nearest cluster center
distances = np.linalg.norm(features[:, np.newaxis, :] - centers, axis=2)
assignments = np.argmin(distances, axis=1)
# Update the cluster centers as the mean of the assigned pixels
for i in range(k):
centers[i] = np.mean(features[assignments == i], axis=0)
return assignments
```
This implementation uses vectorized operations to compute the distances between each pixel and each cluster center, and to update the cluster centers based on the assigned pixels. The `features` input should be an array of shape `(N, 3)` containing the RGB color information of each pixel in the image, and `k` is the desired number of clusters. The function returns an array of length `N` containing the index of the assigned cluster for each pixel.
相关推荐
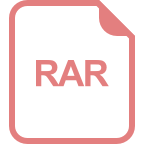















