def use_cuda(enabled, device_id=0):#CUDA检测 if enabled: assert torch.cuda.is_available(), 'CUDA is not available' torch.cuda.set_device(device_id)
时间: 2023-06-06 18:08:26 浏览: 155
这是一个Python函数,用于启用/禁用CUDA加速,并选择使用哪个CUDA设备。如果enabled为True,则启用CUDA加速,并使用device_id指定的设备(默认为0)。如果enabled为False,则禁用CUDA加速。
相关问题
try: import thop except ImportError: thop = None logger = logging.getLogger(__name__) @contextmanager def torch_distributed_zero_first(local_rank: int): if local_rank not in [-1, 0]: torch.distributed.barrier() yield if local_rank == 0: torch.distributed.barrier() def init_torch_seeds(seed=0): torch.manual_seed(seed) if seed == 0: cudnn.benchmark, cudnn.deterministic = False, True else: cudnn.benchmark, cudnn.deterministic = True, False def select_device(device='', batch_size=None): s = f'YOLOv5 🚀 {git_describe() or date_modified()} torch {torch.__version__} ' cpu = device.lower() == 'cpu' if cpu: os.environ['CUDA_VISIBLE_DEVICES'] = '-1' elif device: # non-cpu device requested os.environ['CUDA_VISIBLE_DEVICES'] = device assert torch.cuda.is_available(), f'CUDA unavailable, invalid device {device} requested' cuda = not cpu and torch.cuda.is_available() if cuda: n = torch.cuda.device_count() if n > 1 and batch_size: # check that batch_size is compatible with device_count assert batch_size % n == 0, f'batch-size {batch_size} not multiple of GPU count {n}' space = ' ' * len(s) for i, d in enumerate(device.split(',') if device else range(n)): p = torch.cuda.get_device_properties(i) s += f"{'' if i == 0 else space}CUDA:{d} ({p.name}, {p.total_memory / 1024 ** 2}MB)\n" s += 'CPU\n' logger.info(s.encode().decode('ascii', 'ignore') if platform.system() == 'Windows' else s) # emoji-safe return torch.device('cuda:0' if cuda else 'cpu') def time_synchronized(): if torch.cuda.is_available(): torch.cuda.synchronize() return time.time()
这段代码是一个Python脚本,包含了一些用于PyTorch训练的实用函数。具体来说,它包含了以下函数:
- import thop:导入PyTorch的模块thop,用于计算模型的FLOPs和参数数量。
- torch_distributed_zero_first:用于在分布式训练中同步所有进程的执行顺序。
- init_torch_seeds:用于初始化PyTorch的随机数种子。
- select_device:用于选择PyTorch的设备,可以选择CPU或GPU。
- time_synchronized:用于在CPU和GPU之间同步时间。
这些函数都是用于方便PyTorch训练的实用函数,可以大大简化代码编写。
LDAM损失函数pytorch代码如下:class LDAMLoss(nn.Module): def __init__(self, cls_num_list, max_m=0.5, weight=None, s=30): super(LDAMLoss, self).__init__() m_list = 1.0 / np.sqrt(np.sqrt(cls_num_list)) m_list = m_list * (max_m / np.max(m_list)) m_list = torch.cuda.FloatTensor(m_list) self.m_list = m_list assert s > 0 self.s = s if weight is not None: weight = torch.FloatTensor(weight).cuda() self.weight = weight self.cls_num_list = cls_num_list def forward(self, x, target): index = torch.zeros_like(x, dtype=torch.uint8) index_float = index.type(torch.cuda.FloatTensor) batch_m = torch.matmul(self.m_list[None, :], index_float.transpose(1,0)) # 0,1 batch_m = batch_m.view((16, 1)) # size=(batch_size, 1) (-1,1) x_m = x - batch_m output = torch.where(index, x_m, x) if self.weight is not None: output = output * self.weight[None, :] target = torch.flatten(target) # 将 target 转换成 1D Tensor logit = output * self.s return F.cross_entropy(logit, target, weight=self.weight) 模型部分参数如下:# 设置全局参数 model_lr = 1e-5 BATCH_SIZE = 16 EPOCHS = 50 DEVICE = torch.device('cuda:0' if torch.cuda.is_available() else 'cpu') use_amp = True use_dp = True classes = 7 resume = None CLIP_GRAD = 5.0 Best_ACC = 0 #记录最高得分 use_ema=True model_ema_decay=0.9998 start_epoch=1 seed=1 seed_everything(seed) # 数据增强 mixup mixup_fn = Mixup( mixup_alpha=0.8, cutmix_alpha=1.0, cutmix_minmax=None, prob=0.1, switch_prob=0.5, mode='batch', label_smoothing=0.1, num_classes=classes) 帮我用pytorch实现模型在模型训练中使用LDAM损失函数
好的,以下是使用LDAM损失函数进行模型训练的示例代码:
```python
import torch
import torch.nn as nn
import torch.optim as optim
import torch.nn.functional as F
from ldam_loss import LDAMLoss # 导入LDAM损失函数
# 定义模型
class MyModel(nn.Module):
def __init__(self):
super(MyModel, self).__init__()
self.conv1 = nn.Conv2d(3, 64, 3, padding=1)
self.bn1 = nn.BatchNorm2d(64)
self.conv2 = nn.Conv2d(64, 128, 3, padding=1)
self.bn2 = nn.BatchNorm2d(128)
self.conv3 = nn.Conv2d(128, 256, 3, padding=1)
self.bn3 = nn.BatchNorm2d(256)
self.fc1 = nn.Linear(256 * 4 * 4, 1024)
self.fc2 = nn.Linear(1024, 10)
def forward(self, x):
x = F.relu(self.bn1(self.conv1(x)))
x = F.max_pool2d(x, 2)
x = F.relu(self.bn2(self.conv2(x)))
x = F.max_pool2d(x, 2)
x = F.relu(self.bn3(self.conv3(x)))
x = F.max_pool2d(x, 2)
x = x.view(-1, 256 * 4 * 4)
x = F.relu(self.fc1(x))
x = self.fc2(x)
return x
# 定义数据集和数据加载器
train_dataset = ... # 定义训练集
test_dataset = ... # 定义测试集
train_loader = torch.utils.data.DataLoader(train_dataset, batch_size=16, shuffle=True)
test_loader = torch.utils.data.DataLoader(test_dataset, batch_size=16, shuffle=False)
# 定义LDAM损失函数
cls_num_list = ... # 定义每个类别的样本数量
criterion = LDAMLoss(cls_num_list, max_m=0.5, s=30)
# 定义优化器和学习率调度器
model = MyModel().to('cuda')
optimizer = optim.Adam(model.parameters(), lr=1e-3)
scheduler = optim.lr_scheduler.StepLR(optimizer, step_size=10, gamma=0.1)
# 训练模型
for epoch in range(50):
model.train()
for batch_idx, (data, target) in enumerate(train_loader):
data, target = data.to('cuda'), target.to('cuda')
data, target_a, target_b, lam = mixup_fn(data, target)
optimizer.zero_grad()
output = model(data)
loss = criterion(output, target_a, target_b, lam) # 使用LDAM损失函数
loss.backward()
nn.utils.clip_grad_norm_(model.parameters(), CLIP_GRAD) # 防止梯度爆炸
optimizer.step()
model.eval()
with torch.no_grad():
total = 0
correct = 0
for data, target in test_loader:
data, target = data.to('cuda'), target.to('cuda')
output = model(data)
_, predicted = torch.max(output.data, 1)
total += target.size(0)
correct += (predicted == target).sum().item()
acc = 100 * correct / total
print('Epoch {}: Test Acc = {}%'.format(epoch+1, acc))
scheduler.step()
```
在以上代码中,我们首先定义了一个名为`MyModel`的模型,然后定义了训练集和测试集的数据加载器。接着,我们使用LDAM损失函数来定义模型的损失函数,然后使用Adam优化器来训练模型。在每个epoch结束后,我们使用测试集来评估模型的性能,并使用学习率调度器来调整学习率。
阅读全文
相关推荐
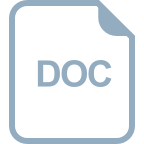
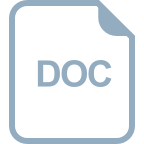
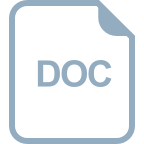











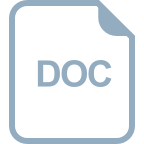
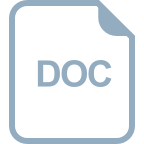