matrix_r = np.random.randint(0, 256, size=(128, 128)) print(matrix_r) matrix_g = np.random.randint(0, 256, size=(128, 128)) matrix_b = np.random.randint(0, 256, size=(128, 128)) print(matrix_b) print(matrix_b.shape) # 将三个矩阵拼接成一个3通道128乘128的矩阵
时间: 2024-03-06 19:48:07 浏览: 20
可以使用numpy中的dstack函数将三个矩阵按深度方向进行拼接,生成一个3通道128x128的矩阵,代码如下:
```
matrix_rgb = np.dstack((matrix_r, matrix_g, matrix_b))
print(matrix_rgb)
print(matrix_rgb.shape)
```
其中,dstack函数的作用是将三个矩阵按深度方向进行拼接,生成一个3通道的矩阵。最后输出拼接后的矩阵以及其形状。
相关问题
matrix_r = np.random.randint(0, 256, size=(128, 128)) print(matrix_r) matrix_g = np.random.randint(0, 256, size=(128, 128)) matrix_b = np.random.randint(0, 256, size=(128, 128)) print(matrix_b) print(matrix_b.shape) # 将三个矩阵拼接成一个3通道128乘128的图片
要将三个矩阵拼接成一个3通道128乘128的图片,可以使用matplotlib库中的imshow函数来实现,代码如下:
```
import matplotlib.pyplot as plt
# 将三个矩阵拼接成一个3通道的矩阵
matrix_rgb = np.dstack((matrix_r, matrix_g, matrix_b))
# 显示图片
plt.imshow(matrix_rgb)
plt.show()
```
其中,dstack函数的作用是将三个矩阵按深度方向进行拼接,生成一个3通道的矩阵。imshow函数的作用是显示图片。最后使用show函数显示图片。
补全程序,完成以下功能:创建表示10×10的随机矩阵的DataFrame 对象,行索引名和列索引名都为1~10,元素取值在0~100,并求每列的最大值和最小值。输出参考如下运行示例:import pandas as pd import ______ as np data = np.random.randint(0, 101, (____, ____), np.int32) index = np.arange(1, 11, 1) matrix = pd.DataFrame(data, ____, index) # 创建DataFrame对象 print(matrix) matrix_max = matrix.____(axis=0) # 求每列最大值 print(matrix_max) matrix_min = matrix.min(axis=____) # 求每列最小值 print(matrix_min)
import pandas as pd
import numpy as np
data = np.random.randint(0, 101, (10, 10), np.int32)
index = np.arange(1, 11, 1)
matrix = pd.DataFrame(data, index, index) # 创建DataFrame对象
print(matrix)
matrix_max = matrix.max(axis=0) # 求每列最大值
print(matrix_max)
matrix_min = matrix.min(axis=0) # 求每列最小值
print(matrix_min)
相关推荐
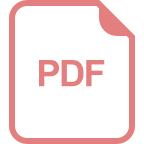
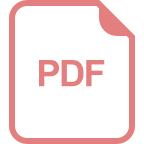














