优化这段代码:def calDistanceMatrix(model): for i in range(len(model.demand_id_list)): from_node_id = model.demand_id_list[i] for j in range(i + 1, len(model.demand_id_list)): to_node_id = model.demand_id_list[j] dist = math.sqrt((model.demand_dict[from_node_id].x_coord - model.demand_dict[to_node_id].x_coord) ** 2 + (model.demand_dict[from_node_id].y_coord - model.demand_dict[to_node_id].y_coord) ** 2) model.distance_matrix[from_node_id, to_node_id] = dist model.distance_matrix[to_node_id, from_node_id] = dist for _, vehicle in model.vehicle_dict.items(): dist = math.sqrt((model.demand_dict[from_node_id].x_coord - vehicle.x_coord) ** 2 + (model.demand_dict[from_node_id].y_coord - vehicle.y_coord) ** 2) model.distance_matrix[from_node_id, vehicle.type] = dist model.distance_matrix[vehicle.type, from_node_id] = dist
时间: 2024-04-01 22:34:03 浏览: 65
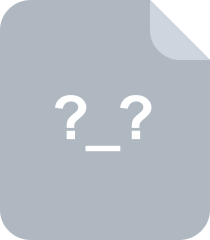
优化模型代码
可以考虑使用numpy来优化这段代码,同时减少重复计算。代码如下:
import numpy as np
def calDistanceMatrix(model):
n = len(model.demand_id_list)
demand_coords = np.array([[model.demand_dict[i].x_coord, model.demand_dict[i].y_coord] for i in model.demand_id_list])
for i in range(n):
from_node_id = model.demand_id_list[i]
demand_coord = demand_coords[i]
for j in range(i + 1, n):
to_node_id = model.demand_id_list[j]
to_demand_coord = demand_coords[j]
dist = np.linalg.norm(demand_coord - to_demand_coord)
model.distance_matrix[from_node_id, to_node_id] = dist
model.distance_matrix[to_node_id, from_node_id] = dist
for _, vehicle in model.vehicle_dict.items():
vehicle_coord = np.array([vehicle.x_coord, vehicle.y_coord])
dist = np.linalg.norm(demand_coord - vehicle_coord)
model.distance_matrix[from_node_id, vehicle.type] = dist
model.distance_matrix[vehicle.type, from_node_id] = dist
这里使用了numpy中的`linalg.norm`函数来计算两点之间的距离,同时利用numpy数组的广播特性,避免了循环计算。
阅读全文
相关推荐
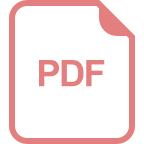
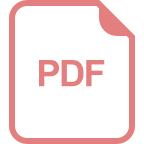













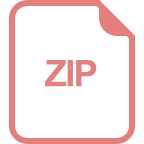
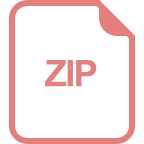
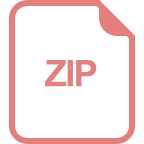