n_examples = X.shape[0]解释代码
时间: 2024-06-02 11:07:31 浏览: 96
这段代码是Python语言中的一个赋值语句,其中X是一个多维数组或矩阵对象,它的shape属性可以返回数组或矩阵的维度信息。其中,X.shape[0]表示X对象的第一维度大小,也即X中样本个数。这个值被赋值给变量n_examples,作为一个标量数值。该代码片段通常出现在机器学习中数据预处理的过程中,用于统计数据集中样本的个数。
相关问题
import os import pickle import cv2 import matplotlib.pyplot as plt import numpy as np from keras.layers import Conv2D, MaxPooling2D, Flatten, Dense, Dropout from keras.models import Sequential from keras.optimizers import adam_v2 from keras_preprocessing.image import ImageDataGenerator from sklearn.model_selection import train_test_split from sklearn.preprocessing import LabelEncoder, OneHotEncoder, LabelBinarizer def load_data(filename=r'/root/autodl-tmp/RML2016.10b.dat'): with open(r'/root/autodl-tmp/RML2016.10b.dat', 'rb') as p_f: Xd = pickle.load(p_f, encoding="latin-1") # 提取频谱图数据和标签 spectrograms = [] labels = [] train_idx = [] val_idx = [] test_idx = [] np.random.seed(2016) a = 0 for (mod, snr) in Xd: X_mod_snr = Xd[(mod, snr)] for i in range(X_mod_snr.shape[0]): data = X_mod_snr[i, 0] frequency_spectrum = np.fft.fft(data) power_spectrum = np.abs(frequency_spectrum) ** 2 spectrograms.append(power_spectrum) labels.append(mod) train_idx += list(np.random.choice(range(a * 6000, (a + 1) * 6000), size=3600, replace=False)) val_idx += list(np.random.choice(list(set(range(a * 6000, (a + 1) * 6000)) - set(train_idx)), size=1200, replace=False)) a += 1 # 数据预处理 # 1. 将频谱图的数值范围调整到0到1之间 spectrograms_normalized = spectrograms / np.max(spectrograms) # 2. 对标签进行独热编码 label_binarizer = LabelBinarizer() labels_encoded= label_binarizer.fit_transform(labels) # transfor the label form to one-hot # 3. 划分训练集、验证集和测试集 # X_train, X_temp, y_train, y_temp = train_test_split(spectrograms_normalized, labels_encoded, test_size=0.15, random_state=42) # X_val, X_test, y_val, y_test = train_test_split(X_temp, y_temp, test_size=0.5, random_state=42) spectrogramss = np.array(spectrograms_normalized) print(spectrogramss.shape) labels = np.array(labels) X = np.vstack(spectrogramss) n_examples = X.shape[0] test_idx = list(set(range(0, n_examples)) - set(train_idx) - set(val_idx)) np.random.shuffle(train_idx) np.random.shuffle(val_idx) np.random.shuffle(test_idx) X_train = X[train_idx] X_val = X[val_idx] X_test = X[test_idx] print(X_train.shape) print(X_val.shape) print(X_test.shape) y_train = labels[train_idx] y_val = labels[val_idx] y_test = labels[test_idx] print(y_train.shape) print(y_val.shape) print(y_test.shape) # X_train = np.expand_dims(X_train,axis=-1) # X_test = np.expand_dims(X_test,axis=-1) # print(X_train.shape) return (mod, snr), (X_train, y_train), (X_val, y_val), (X_test, y_test) 这是我的数据预处理代码
你的数据预处理代码看起来很完整。它首先加载pickle文件,然后提取频谱图数据和标签。接下来,它对频谱图数据进行归一化处理,并对标签进行独热编码。最后,它将数据集划分为训练集、验证集和测试集,并返回这些数据集。
在代码中,你还有一些注释掉的代码段,例如对数据集进行划分的部分。如果你打算使用这些划分代码,你可以取消注释相应的行。另外,如果你的频谱图数据维度是二维的,你可能需要使用`np.expand_dims`函数将其扩展为三维,以便与Conv2D层兼容。
如果你还有其他问题或需要进一步帮助,请随时问我。
img = imread(r'I:\\18Breakageratecalculation\\mask-slic use\\maskSLIC-master\\1\\056.jpg') # The ROI is also stored as an image for viewing convenience # But the roi input input maskSLIC should be a binary image with the same spatial # Dimensions as the image (in this case 300x451) roi = imread(r'I:\\18Breakageratecalculation\\mask-slic use\\maskSLIC-master\\1\\0562.png') # The alpha channel is used to store the ROI in this case and is converted into a logical array of 0s and 1s roi = roi[:, :, 3] > 0 # Alternatively a mask could be created manually with for example a disk: # roi = np.zeros((img.shape[0], img.shape[1])) # a, b = 150, 150 # r = 100 # y,x = np.ogrid[-a:img.shape[0]-a, -b:img.shape[1]-b] # mask = x*x + y*y <= r*r # roi[mask] = 1 # ~~~~~~~~~~~~ Example 1: maskSLIC ~~~~~~~~~~~~~ t1 = time.time() # Note that compactness is defined differently because a grid is not used. Lower compactness for maskSLIC is equivalent segments = seg.slic(img, compactness=10, seed_type='nplace', mask=roi, n_segments=120, recompute_seeds=True, plot_examples=True, enforce_connectivity=True) print("Time: {:.2f} s".format(time.time() - t1)) plt.figure() plt.imshow(mark_boundaries(img, segments)) plt.contour(roi, contours=1, colors='red', linewidths=0.5) plt.axis('off') # ~~~~~~~~~~~ Example 2: SLIC ~~~~~~~~~~~~~~~~~ t1 = time.time() segments = seg.slic(img, compactness=10, seed_type='grid', n_segments=100, plot_examples=False, enforce_connectivity=True) # segments[roi==0] = -1 print("Time: {:.2f} s".format(time.time() - t1)) plt.figure() plt.imshow(mark_boundaries(img, segments)) plt.contour(roi, contours=1, colors='red', linewidths=1) plt.axis('off') plt.show() plt.show()怎么保存结果
如果想要保存分割结果,可以使用matplotlib库中的imsave函数来保存图像。
例如,可以在代码中添加以下语句来保存Example 1中的分割结果:
```
plt.imshow(mark_boundaries(img, segments))
plt.contour(roi, contours=1, colors='red', linewidths=0.5)
plt.axis('off')
plt.savefig('example1.png') # 保存图片
plt.show()
```
这样就会在当前路径下生成一个名为example1.png的图片文件,其中包含分割结果和ROI边界。需要保存Example 2的分割结果时,也可以类似地添加保存语句。
阅读全文
相关推荐
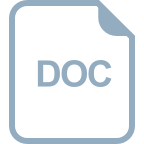
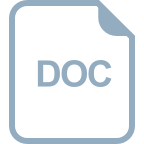
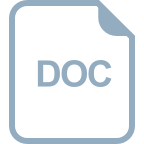


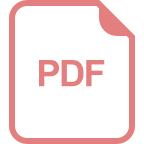










