如何利用python的REF-RF进行遥感图像特征筛选
时间: 2023-08-10 14:07:05 浏览: 178
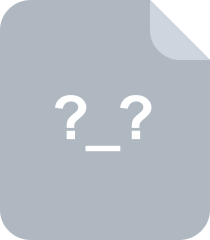
python读取遥感影像

Remote sensing image feature selection using Python's REF-RF can be done using the following steps:
1. Install the required packages - scikit-learn, numpy, gdal, and rasterio.
2. Load the remote sensing image using gdal or rasterio and convert it into a numpy array.
3. Create a pandas dataframe with the numpy array as input and the class labels as output.
4. Split the dataset into training and testing sets.
5. Use the Recursive Feature Elimination (RFE) function from scikit-learn to select the most important features.
6. Use the Random Forest classifier to train the model on the reduced feature set.
7. Evaluate the performance of the model on the testing set.
Here is some example code to get you started:
```python
import numpy as np
import pandas as pd
from sklearn.ensemble import RandomForestClassifier
from sklearn.feature_selection import RFE
from sklearn.model_selection import train_test_split
import rasterio
# Load the remote sensing image
with rasterio.open('remote_sensing_image.tif') as src:
image = src.read()
# Convert the image into a numpy array
image = np.array(image)
# Load the class labels
class_labels = pd.read_csv('class_labels.csv')
# Create a pandas dataframe with the image as input and class labels as output
data = pd.DataFrame({'features': image.reshape((image.shape[0]*image.shape[1]), image.shape[2]), 'class': class_labels})
# Split the dataset into training and testing sets
X_train, X_test, y_train, y_test = train_test_split(data['features'], data['class'], test_size=0.3)
# Use RFE to select the most important features
rfc = RandomForestClassifier(n_estimators=100)
rfe = RFE(estimator=rfc, n_features_to_select=10, step=1)
rfe.fit(X_train, y_train)
# Train the model on the reduced feature set
rfc.fit(rfe.transform(X_train), y_train)
# Evaluate the performance of the model on the testing set
accuracy = rfc.score(rfe.transform(X_test), y_test)
print("Accuracy:", accuracy)
```
In the above code, we train a Random Forest classifier on the reduced feature set selected by RFE. The number of features to select can be adjusted by changing the `n_features_to_select` parameter.
阅读全文
相关推荐
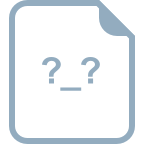
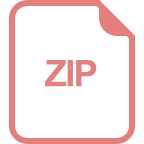
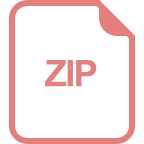
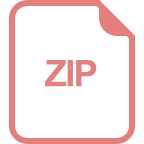
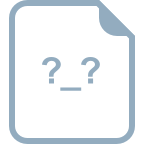
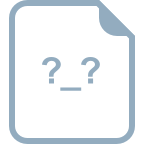
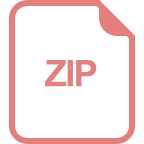
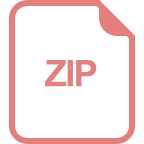
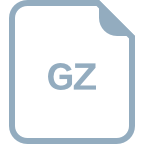
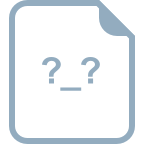
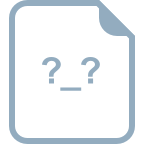
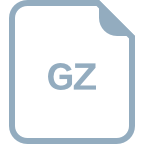
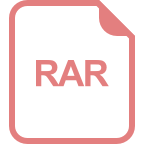