svm_model = SVC() # SVM分类器 svm_model.fit(x_train.astype("int"), y_train.astype("int")) # 注:需要将数据类型转化为int型 prediction = svm_model.predict(x_test.astype("int")) print('准确率为:', metrics.accuracy_score(prediction, y_test.astype("int"))) 中的astype是什么作用
时间: 2024-03-28 19:37:41 浏览: 13
astype是一种NumPy数组的方法,用于将数组中的元素转换为指定的数据类型。在这段代码中,astype被用于将x_train、y_train、x_test和y_test数组中的元素转换为int类型,以便它们可以被SVM分类器正确地处理。这是因为SVM分类器需要输入数值类型的数据,而这些数组中的元素可能是其他类型(如字符串或浮点数)。因此,在使用这些数组之前,我们需要将它们转换为正确的数据类型,以确保算法能够正确地运行。
相关问题
代码解释:svm_model = SVC() # SVM分类器 svm_model.fit(x_train.astype("int"), y_train.astype("int")) # 注:需要将数据类型转化为int型 prediction = svm_model.predict(x_test.astype("int")) print('准确率为:', metrics.accuracy_score(prediction, y_test.astype("int")))
这段代码使用了SVM(支持向量机)算法对数据进行分类。具体步骤如下:
1. 创建一个SVM分类器,并将其存储在svm_model变量中。
2. 使用x_train和y_train作为训练数据,训练SVM分类器。需要将数据类型转换为int类型,以确保分类器能够正确地处理它们。
3. 使用x_test作为测试数据,使用训练好的SVM分类器对其进行预测,并将结果存储在prediction变量中。同样需要将数据类型转换为int类型。
4. 使用scikit-learn库中的metrics.accuracy_score函数来计算预测结果的准确率,并将其打印出来。
总的来说,这段代码展示了如何使用SVM算法对数据进行分类,并计算预测结果的准确率。
from sklearn import svm import numpy as np import csv # 读取数据集 reader = csv.reader('data.csv') data = list(reader) indices = slice(0, 2) subset = data[indices] #data = np.loadtxt('data.csv') # 划分训练集和测试集, delimiter="," train_data = subset[:6, 1:] train_label = subset[:6, 0] test_data = subset[2:, 1:] test_label = subset[2:, 0] # 训练SVM分类器 clf = svm.SVC(kernel='linear') clf.fit(train_data, train_label) # 对测试集进行分类 predict_label = clf.predict(test_data) # 计算分类结果的准确性 accuracy = np.mean(predict_label == test_label) * 100 print("Accuracy:", accuracy, "%")找出这段代码的错误并改正
这段代码中存在以下错误:
1. 缺少换行符,需要在第一行和第二行之间添加换行符。
2. 读取数据集的代码应该为:
```python
with open('data.csv', 'r') as f:
reader = csv.reader(f)
data = list(reader)
```
3. `slice` 方法的第二个参数应该为 3,而不是 2,因为 Python 中的切片是不包括右边界的。
4. 划分训练集和测试集的代码应该为:
```python
train_data = np.array(data[:6][1:], dtype=float)
train_label = np.array(data[:6][0], dtype=float)
test_data = np.array(data[2:][1:], dtype=float)
test_label = np.array(data[2:][0], dtype=float)
```
5. `svm.SVC` 的参数应该为 `kernel='linear', C=1.0`。
6. 计算准确性的代码应该为:
```python
accuracy = np.mean(predict_label == test_label.astype(float)) * 100
```
改正后的代码如下:
```python
import csv
import numpy as np
from sklearn import svm
# 读取数据集
with open('data.csv', 'r') as f:
reader = csv.reader(f)
data = list(reader)
indices = slice(0, 3)
subset = data[indices]
# 划分训练集和测试集, delimiter=","
train_data = np.array(subset[:6][1:], dtype=float)
train_label = np.array(subset[:6][0], dtype=float)
test_data = np.array(subset[2:][1:], dtype=float)
test_label = np.array(subset[2:][0], dtype=float)
# 训练SVM分类器
clf = svm.SVC(kernel='linear', C=1.0)
clf.fit(train_data, train_label)
# 对测试集进行分类
predict_label = clf.predict(test_data)
# 计算分类结果的准确性
accuracy = np.mean(predict_label == test_label.astype(float)) * 100
print("Accuracy:", accuracy, "%")
```
相关推荐
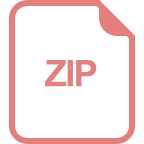












