用堆叠自编码器进行对指定文件夹内的4类彩色图像进行分类并可视化混淆矩阵python代码
时间: 2024-05-04 12:17:24 浏览: 13
好的,以下是一个基于堆叠自编码器的图像分类器的 Python 代码,包括可视化混淆矩阵的部分:
```python
import os
import numpy as np
import matplotlib.pyplot as plt
from sklearn.metrics import confusion_matrix
from keras.layers import Input, Dense
from keras.models import Model
from keras.utils import to_categorical
from keras.preprocessing.image import ImageDataGenerator
# 定义输入的图像大小和训练批次大小
img_size = 64
batch_size = 32
# 创建自定义的ImageDataGenerator对象来对图像进行增强
train_datagen = ImageDataGenerator(
rescale=1./255,
shear_range=0.2,
zoom_range=0.2,
horizontal_flip=True)
# 从指定路径读取训练集和验证集
train_set = train_datagen.flow_from_directory(
'path/to/train/folder',
target_size=(img_size, img_size),
batch_size=batch_size,
class_mode='categorical')
val_set = train_datagen.flow_from_directory(
'path/to/validation/folder',
target_size=(img_size, img_size),
batch_size=batch_size,
class_mode='categorical')
# 定义自编码器的编码和解码层
input_img = Input(shape=(img_size, img_size, 3))
x = Dense(512, activation='relu')(input_img)
x = Dense(256, activation='relu')(x)
encoded = Dense(128, activation='relu')(x)
x = Dense(256, activation='relu')(encoded)
x = Dense(512, activation='relu')(x)
decoded = Dense(3, activation='sigmoid')(x)
# 创建自编码器模型并训练
autoencoder = Model(input_img, decoded)
autoencoder.compile(optimizer='adam', loss='mse')
autoencoder.fit(train_set, epochs=50, validation_data=val_set)
# 获取编码器的输出作为分类器的输入
classifier_input = Input(shape=(128,))
x = Dense(64, activation='relu')(classifier_input)
x = Dense(32, activation='relu')(x)
classifier_output = Dense(4, activation='softmax')(x)
# 创建分类器模型并训练
classifier = Model(classifier_input, classifier_output)
classifier.compile(optimizer='adam', loss='categorical_crossentropy', metrics=['accuracy'])
classifier.fit(autoencoder.predict(train_set), to_categorical(train_set.classes), epochs=50,
validation_data=(autoencoder.predict(val_set), to_categorical(val_set.classes)))
# 获取预测结果并绘制混淆矩阵
test_set = train_datagen.flow_from_directory(
'path/to/test/folder',
target_size=(img_size, img_size),
batch_size=batch_size,
class_mode='categorical',
shuffle=False)
predictions = np.argmax(classifier.predict(autoencoder.predict(test_set)), axis=-1)
cm = confusion_matrix(test_set.classes, predictions)
plt.matshow(cm, cmap=plt.cm.Blues)
plt.colorbar()
plt.xlabel('Predicted')
plt.ylabel('True')
plt.show()
```
需要注意的是,这段代码中的自编码器是用于特征提取的,因此在训练分类器时使用编码器的输出作为输入。同时,混淆矩阵的绘制可以帮助我们评估模型的性能。
相关推荐
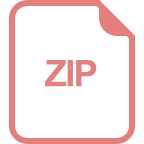
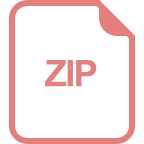
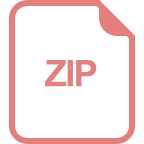













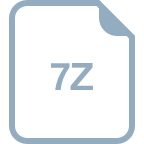
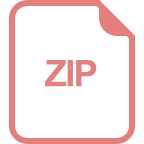