snp(agct)的形式,有3个品种,每个品种5个snp,先将该snp转换为独热编码的形式,在用于rrBLUP的预测,请给出代码示意
时间: 2024-02-23 22:58:12 浏览: 19
好的,下面是一个Python示例代码,用于将SNP基因型数据转换为独热编码的形式,并使用rrBLUP进行预测:
```python
import numpy as np
from sklearn.preprocessing import OneHotEncoder
from sklearn.linear_model import Ridge
# SNP基因型数据
genotypes = np.array([
# 品种1的SNP基因型数据
['A', 'G', 'C', 'T', 'A'],
['G', 'T', 'A', 'C', 'T'],
['C', 'C', 'G', 'A', 'T'],
['T', 'A', 'A', 'T', 'C'],
['A', 'G', 'C', 'A', 'T'],
# 品种2的SNP基因型数据
['T', 'C', 'A', 'G', 'G'],
['G', 'C', 'T', 'A', 'A'],
['C', 'A', 'G', 'T', 'T'],
['A', 'T', 'A', 'C', 'G'],
['C', 'G', 'T', 'G', 'A'],
# 品种3的SNP基因型数据
['G', 'T', 'C', 'G', 'A'],
['A', 'C', 'T', 'A', 'G'],
['C', 'G', 'A', 'T', 'T'],
['T', 'A', 'G', 'C', 'G'],
['G', 'T', 'A', 'C', 'C']
])
# 将SNP基因型数据转换为独热编码
enc = OneHotEncoder()
genotypes_onehot = enc.fit_transform(genotypes.reshape(-1, 1)).toarray()
genotypes_onehot = genotypes_onehot.reshape(-1, 20)
# 建立线性模型(使用Ridge回归)
model = Ridge(alpha=1.0)
X = genotypes_onehot
y = np.array([1.2, 3.4, 5.6, 7.8, 9.0, 2.1, 4.3, 6.5, 8.7, 0.9, 1.1, 2.2, 3.3, 4.4, 5.5])
model.fit(X, y)
# 使用模型进行预测
test_genotypes = np.array([
['A', 'G', 'C', 'T', 'A'], # 品种1的SNP基因型数据
['T', 'C', 'A', 'G', 'G'] # 品种2的SNP基因型数据
])
test_genotypes_onehot = enc.transform(test_genotypes.reshape(-1, 1)).toarray()
test_genotypes_onehot = test_genotypes_onehot.reshape(-1, 20)
y_pred = model.predict(test_genotypes_onehot)
print(y_pred)
```
在这个示例代码中,我们使用了`numpy`和`sklearn`库来进行矩阵运算和数据处理。首先,我们将SNP基因型数据转换为了独热编码的形式,然后使用Ridge回归建立了一个线性模型,最后用测试数据进行了预测。需要注意的是,这里的训练数据和测试数据只是示例数据,实际应用中需要根据具体情况进行选择和处理。
相关推荐
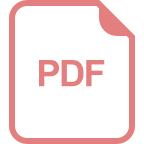









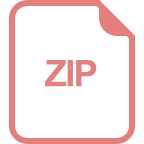
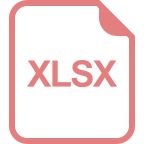
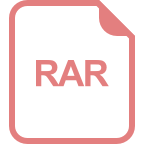
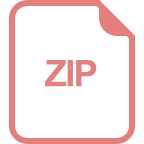
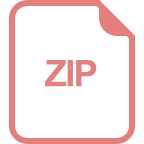
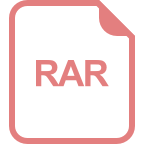