cnn模型python处理数值
时间: 2023-08-13 10:09:14 浏览: 141
CNN(卷积神经网络)是一种用于图像处理和分类的深度学习模型。在Python中,可以使用许多库来实现CNN模型。其中最常用的是TensorFlow和Keras。
在CNN模型中,输入数据通常是图像像素值的矩阵,这些值可以通过Python中的NumPy库来表示。然后,可以使用TensorFlow或Keras来构建CNN模型,并将这些像素值矩阵作为输入数据。
在CNN模型中,卷积层、池化层和全连接层等层级可以使用TensorFlow或Keras中的相应函数来实现。这些函数可以接受像素值矩阵作为输入,并根据模型的配置对其进行处理。
在训练CNN模型时,可以使用Python中的各种优化器和损失函数来训练模型。例如,可以使用TensorFlow中的Adam优化器和交叉熵损失函数来训练CNN模型。
总之,在Python中处理数值数据,可以使用NumPy库和深度学习框架(如TensorFlow和Keras)来构建和训练CNN模型。
相关问题
基于小数据集的,图像和数值数据作为输入的实现多分类的CNN模型,其中数值数据和图像数据在全连接层融合,生成代码
好的,以下是一个基于Keras框架实现的CNN多分类模型的代码,该模型接受图像和数值数据作为输入,并在全连接层中将它们融合:
```python
from keras.models import Model
from keras.layers import Input, Dense, Dropout, Flatten, Conv2D, MaxPooling2D, concatenate
from keras.optimizers import Adam
from keras.utils import to_categorical
import numpy as np
# 加载数据
x_image = np.load('image_data.npy')
x_numeric = np.load('numeric_data.npy')
y = np.load('labels.npy')
# 数据预处理
x_image = x_image.astype('float32') / 255.0 # 归一化
x_numeric = x_numeric.astype('float32')
y = to_categorical(y) # 转换为one-hot编码
# 构建模型
input_image = Input(shape=(28, 28, 3))
conv1 = Conv2D(32, kernel_size=(3, 3), activation='relu')(input_image)
conv2 = Conv2D(64, kernel_size=(3, 3), activation='relu')(conv1)
pooling = MaxPooling2D(pool_size=(2, 2))(conv2)
dropout1 = Dropout(0.25)(pooling)
flatten1 = Flatten()(dropout1)
input_numeric = Input(shape=(10,))
dense1 = Dense(128, activation='relu')(input_numeric)
dropout2 = Dropout(0.5)(dense1)
flatten2 = Flatten()(dropout2)
concat = concatenate([flatten1, flatten2]) # 将两个特征向量合并
dense2 = Dense(64, activation='relu')(concat)
dropout3 = Dropout(0.5)(dense2)
output = Dense(3, activation='softmax')(dropout3) # 多分类问题,输出层使用softmax激活函数
model = Model(inputs=[input_image, input_numeric], outputs=output)
# 编译模型
model.compile(loss='categorical_crossentropy', optimizer=Adam(lr=0.001), metrics=['accuracy'])
# 训练模型
model.fit([x_image, x_numeric], y, batch_size=32, epochs=10, validation_split=0.2)
# 评估模型
score = model.evaluate([x_image, x_numeric], y)
print('Test loss:', score[0])
print('Test accuracy:', score[1])
```
说明:
- 数据集包含图像数据和数值数据,分别存储在`image_data.npy`和`numeric_data.npy`中;
- 标签数据存储在`labels.npy`中,使用`to_categorical`函数将其转换为one-hot编码;
- 模型包含一个卷积层和一个池化层,两个全连接层,使用了Dropout技术来防止过拟合;
- 在第一个全连接层中,将数值数据作为输入,使用Flatten将其展开为一个特征向量;
- 在第一个全连接层的输出和第二个全连接层的输入之间,使用`concatenate`函数将两个特征向量合并;
- 输出层使用softmax激活函数,损失函数使用交叉熵,优化器使用Adam,评估指标为准确率;
- 训练过程中将图像数据和数值数据作为输入,使用了20%的数据作为验证集;
- 训练结束后,使用测试集评估模型性能。
在应用 CNN实现预测过程中,首先,对图像和数值数据进行处理;然后,搭建CNN模型,其中图像数据和数值数据采用Transformer进行融合;最后训练并测试模型。生成pytorch代码
好的,以下是一个简单的示例代码:
```python
import torch
import torch.nn as nn
import torch.optim as optim
from torch.utils.data import DataLoader
from torchvision.datasets import CIFAR10
from torchvision.transforms import transforms
# 定义数据处理
transform = transforms.Compose([
transforms.ToTensor(),
transforms.Normalize((0.5, 0.5, 0.5), (0.5, 0.5, 0.5)),
])
# 加载数据
train_set = CIFAR10(root='./data', train=True, download=True, transform=transform)
test_set = CIFAR10(root='./data', train=False, download=True, transform=transform)
train_loader = DataLoader(train_set, batch_size=32, shuffle=True)
test_loader = DataLoader(test_set, batch_size=32, shuffle=False)
# 定义模型
class CNN(nn.Module):
def __init__(self):
super(CNN, self).__init__()
self.conv1 = nn.Conv2d(3, 10, kernel_size=5)
self.conv2 = nn.Conv2d(10, 20, kernel_size=5)
self.pool = nn.MaxPool2d(2)
self.fc1 = nn.Linear(20 * 5 * 5, 50)
def forward(self, x):
x = F.relu(self.conv1(x))
x = self.pool(x)
x = F.relu(self.conv2(x))
x = self.pool(x)
x = x.view(-1, 20 * 5 * 5)
x = F.relu(self.fc1(x))
return x
class Transformer(nn.Module):
def __init__(self):
super(Transformer, self).__init__()
self.encoder_layer = nn.TransformerEncoderLayer(d_model=50, nhead=5)
self.transformer_encoder = nn.TransformerEncoder(self.encoder_layer, num_layers=2)
def forward(self, x):
x = self.transformer_encoder(x)
return x
class ImageRegression(nn.Module):
def __init__(self):
super(ImageRegression, self).__init__()
self.cnn = CNN()
self.transformer = Transformer()
self.regression = nn.Linear(50*2, 1)
def forward(self, image, numerical):
image_feature = self.cnn(image)
numerical_feature = self.transformer(numerical)
fused_feature = torch.cat((image_feature, numerical_feature), dim=1)
output = self.regression(fused_feature)
return output
# 定义训练函数
def train(model, optimizer, criterion):
model.train()
train_loss = 0.0
correct = 0
total = 0
for batch_idx, (inputs, targets) in enumerate(train_loader):
image_inputs, numerical_inputs = inputs[:, :3, :, :], inputs[:, 3:, :, :]
optimizer.zero_grad()
outputs = model(image_inputs, numerical_inputs)
loss = criterion(outputs, targets.float())
loss.backward()
optimizer.step()
train_loss += loss.item()
_, predicted = torch.max(outputs.data, 1)
total += targets.size(0)
correct += predicted.eq(targets).sum().item()
return train_loss/(batch_idx+1), 100.*correct/total
# 定义测试函数
def test(model, criterion):
model.eval()
test_loss = 0.0
correct = 0
total = 0
with torch.no_grad():
for batch_idx, (inputs, targets) in enumerate(test_loader):
image_inputs, numerical_inputs = inputs[:, :3, :, :], inputs[:, 3:, :, :]
outputs = model(image_inputs, numerical_inputs)
loss = criterion(outputs, targets.float())
test_loss += loss.item()
_, predicted = torch.max(outputs.data, 1)
total += targets.size(0)
correct += predicted.eq(targets).sum().item()
return test_loss/(batch_idx+1), 100.*correct/total
# 训练模型
model = ImageRegression()
criterion = nn.MSELoss()
optimizer = optim.Adam(model.parameters(), lr=0.001)
for epoch in range(10):
train_loss, train_acc = train(model, optimizer, criterion)
test_loss, test_acc = test(model, criterion)
print('Epoch: %d, Train Loss: %.3f, Train Acc: %.3f, Test Loss: %.3f, Test Acc: %.3f' % (
epoch+1, train_loss, train_acc, test_loss, test_acc))
```
在这个示例中,我们首先定义了一个包括图像数据和数值数据的数据集,并使用DataLoader将其加载到模型中。然后,我们定义了一个简单的CNN模型和一个Transformer模型,将它们融合在一起,并通过一个线性层对它们进行回归预测。最后,我们使用MSELoss作为损失函数,Adam作为优化器训练模型,并在每个epoch结束时输出训练和测试的loss和accuracy。
阅读全文
相关推荐
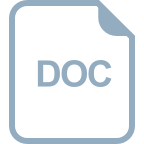
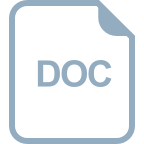
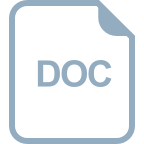
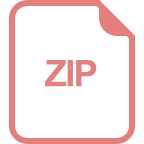
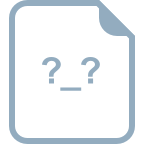
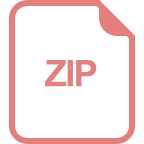
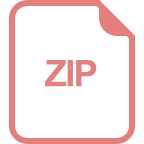
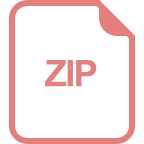
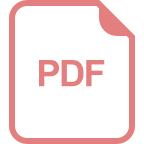
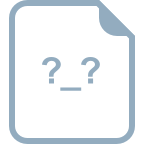
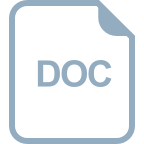
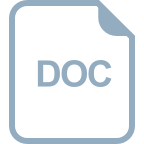
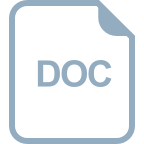



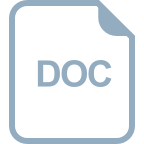