fpr = dict() tpr = dict() roc_auc = dict()
时间: 2024-05-21 08:15:10 浏览: 93
这些代码是用于绘制ROC曲线和计算AUC值的,其中fpr代表false positive rate,tpr代表true positive rate,roc_auc代表ROC曲线下的面积(AUC值)。这些变量都是字典类型,可以通过不同的键来存储不同的数据。在计算ROC曲线和AUC值时,需要根据不同的阈值来计算出对应的fpr和tpr,然后在不同的阈值下计算出ROC曲线下的面积。
相关问题
fpr = dict() tpr = dict() roc_auc = dict() for i in range(3): # 遍历三个类别 fpr[i], tpr[i], _ = roc_curve(y_test[:, i], y_pred[:, i]) roc_auc[i] = auc(fpr[i], tpr[i])出现 too many indices for array: array is 1-dimensional, but 2 were indexed的问题怎么修正
如果您在运行以上代码时出现了 "too many indices for array: array is 1-dimensional, but 2 were indexed" 错误,通常是因为 y_test 和/或 y_pred 的维度不正确。
在这里,y_test 和 y_pred 应该都是二元矩阵(每一列对应一个类别),因此在使用 roc_curve 函数时,需要指定要计算的类别的索引。如果 y_test 和 y_pred 的维度不正确,则可能会出现上述错误。
以下是可能会导致该错误的一些常见原因和解决方法:
- 如果 y_test 和 y_pred 的维度不正确,例如它们是一维数组而不是二元矩阵,则可以使用 label_binarize 函数将其转换为二元矩阵。例如:
```python
from sklearn.preprocessing import label_binarize
# 假设 y_test 和 y_pred 已经定义好
n_classes = len(np.unique(y_test))
binarized_y_test = label_binarize(y_test, classes=range(n_classes))
binarized_y_pred = label_binarize(y_pred, classes=range(n_classes))
```
- 如果 y_test 和 y_pred 的维度正确,但是在使用 roc_curve 函数时出现了错误,则可能是由于指定的类别索引超出了范围。在这种情况下,您应该检查 y_test 和 y_pred 中的类别数量是否与 roc_curve 函数期望的数量匹配,以及类别索引是否正确。例如,如果 y_test 和 y_pred 具有三个类别,则应该将 for 循环的范围更改为 range(3),而不是 range(2)。
在修正以上问题之后,您应该能够成功计算每个类别的 FPR、TPR 和 AUC 值。
y_score = np.array(y_score).T # 计算并绘制 AUC 曲线 fpr = dict() tpr = dict() roc_auc = dict() for i in range(y_test.shape[1]): fpr[i], tpr[i], _ = roc_curve(y_test[:, i], y_score[0, :, i])怎么修正
根据你提供的代码,我看到 y_score 是一个三维数组,它的第一维是样本数,第二维是类别数,第三维是阈值。而在计算 ROC 曲线时,只需要使用 y_score 的第二维和第三维,即每个类别在不同阈值下的得分。因此,你需要修改代码如下:
```python
y_score = np.array(y_score) # 去掉转置操作
fpr = dict()
tpr = dict()
roc_auc = dict()
for i in range(y_test.shape[1]):
fpr[i], tpr[i], _ = roc_curve(y_test[:, i], y_score[:, i, :].ravel()) # 传入每个类别的得分
roc_auc[i] = auc(fpr[i], tpr[i]) # 计算 AUC
```
这里,我们将 y_score 的第二维作为循环变量 i,即每次计算一个类别的 ROC 曲线和 AUC 值。在计算 ROC 曲线时,我们需要将 y_score 的第一维和第三维展开成一维数组,即使用 ravel() 方法。最后,我们将每个类别的 AUC 值存储在字典 roc_auc 中。
阅读全文
相关推荐
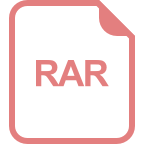
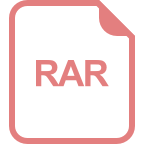
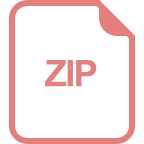













