model = net model.train() model.to(device)是什么意思
时间: 2024-06-14 08:03:44 浏览: 15
根据提供的引用内容,可以看出这是使用Ultralytics YOLOv8进行目标检测模型训练的代码。其中,`model = net`是将模型赋值给变量`net`,`model.train()`是启动模型训练,`model.to(device)`是将模型移动到指定的设备上(例如GPU)。
具体来说,`model = net`是将YOLOv8模型赋值给变量`net`,`model.train()`是启动模型训练,`model.to(device)`是将模型移动到指定的设备上(例如GPU)。这些代码都是在使用Ultralytics YOLOv8进行目标检测模型训练时常用的操作。
相关问题
model = Net(num_features=train_dataset.num_features, dim=dim, num_classes=torch.max(train_dataset.y).item() + 1).to(device)
This code initializes a neural network model with the following parameters:
- `num_features`: The number of input features for the model, which is the number of columns in the input data. This is obtained from the `train_dataset.num_features` attribute.
- `dim`: The dimensionality of the model's hidden layers. This is a hyperparameter that can be set by the user.
- `num_classes`: The number of output classes for the model, which is the maximum value in the `train_dataset.y` attribute plus one. This assumes that the class labels are contiguous integers starting from zero.
- `device`: The device (e.g. CPU or GPU) on which the model will be trained and run.
The `Net` class is assumed to be defined elsewhere in the code, and it should inherit from the `nn.Module` class in PyTorch. The `to` method is used to move the model parameters to the specified device.
yolo task=detect mode=train model=yolov8n.pt data=./data/rbc.yaml batch=32 epochs=100 imgsz=640 workers=4 device=0
根据你提供的信息,这是一个使用YOLOv8n.pt模型在训练模式下进行红细胞检测的命令。以下是该命令的参数解释:
- task=detect:检测任务
- mode=train:训练模式
- model=yolov8n.pt:使用YOLOv8n.pt模型
- data=./data/rbc.yaml:数据配置文件路径
- batch=32:批处理大小为32
- epochs=100:训练轮数为100
- imgsz=640:输入图像大小为640 x 640
- workers=4:使用4个工作线程来加载数据
- device=0:使用第一个GPU设备进行训练
如果你需要更具体的介绍,可以提供更多上下文信息,我将尽力回答。另外,请问还有什么其他的问题或者需要我解答的内容吗?
相关推荐
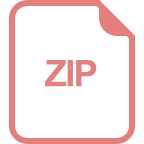
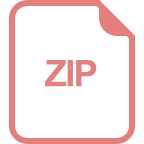
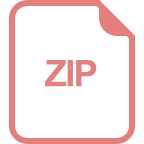












