python function reduce and reduce_forward.
时间: 2023-12-12 16:35:13 浏览: 41
根据提供的引用内容,没有涉及到Python中的reduce和reduce_forward函数。但是,如果您想了解Python中的reduce函数,可以参考以下内容:
reduce函数是Python内置的一个高阶函数,它接收一个函数和一个序列作为参数,然后把这个函数作用在序列上,得到一个结果。reduce函数的具体实现需要导入functools模块。
以下是reduce函数的语法:
```python
functools.reduce(function, iterable[, initializer])
```
其中,function是一个有两个参数的函数,iterable是一个可迭代对象,initializer是可选的初始值。
reduce函数的作用是对序列中的元素进行累积操作,例如求和、求积等。以下是一个求和的例子:
```python
from functools import reduce
lst = [1, 2, 3, 4, 5]
result = reduce(lambda x, y: x + y, lst)
print(result) # 输出:15
```
以上代码中,reduce函数对lst中的元素进行累积求和,最终得到结果15。
相关问题
Write a code to calculate the trajectory that is traced out between time t=0 and t=20 by an air parcel in the flow: F(x, y)=-(y^2)/2+cos(x). Use the explicit (Euler forward) scheme for the first step, and the leapfrog scheme for all subsequent steps. Use △t = 0.01 and include a Robert-Asselin filter applied to both x and y with a coefficient of 0.01. You will need to import math to use the Python function math.sin(). Run your program for the following two starting positions, which are marked with crosses in the above figure:(x0.y0)=(1.0,1.0) (in the eddy region) ,(x0,y0)=(1.0,2.0) (in the stream region) For each of these starting positions, plot the trajectory in the (x ,y) plane. Use the trajectory in the eddy region to verify the stability of the numerical method and explain your reasoning
Sure, here's a Python code that solves the problem you presented:
```python
import math
import matplotlib.pyplot as plt
# Define the flow field function F(x, y)
def F(x, y):
return -(y**2)/2 + math.cos(x)
# Define initial positions
x0, y0 = 1.0, 1.0 # starting position in the eddy region
x1, y1 = 1.0, 2.0 # starting position in the stream region
# Define time step and total time
dt = 0.01
T = 20
# Define Robert-Asselin filter coefficient
alpha = 0.01
# Define arrays to store positions
x_traj = [x0]
y_traj = [y0]
# Solve for starting position (x0, y0)
x, y = x0, y0
for t in range(1, int(T/dt)+1):
# Explicit Euler forward scheme for the first step
if t == 1:
x += dt * F(x, y)
y += dt * F(x0+dt, y)
# Leapfrog scheme for all subsequent steps
else:
x += 2*dt * F(x, y)
y += 2*dt * F(x, y0+dt)
# Apply Robert-Asselin filter
x = x + alpha * (x - x0)
y = y + alpha * (y - y0)
# Store positions
x_traj.append(x)
y_traj.append(y)
# Plot trajectory for starting position (x0, y0)
plt.plot(x_traj, y_traj, label='Starting position: (1.0, 1.0)')
# Repeat for starting position (x1, y1)
x_traj = [x1]
y_traj = [y1]
x, y = x1, y1
for t in range(1, int(T/dt)+1):
if t == 1:
x += dt * F(x, y)
y += dt * F(x1+dt, y)
else:
x += 2*dt * F(x, y)
y += 2*dt * F(x, y1+dt)
x = x + alpha * (x - x1)
y = y + alpha * (y - y1)
x_traj.append(x)
y_traj.append(y)
# Plot trajectory for starting position (x1, y1)
plt.plot(x_traj, y_traj, label='Starting position: (1.0, 2.0)')
# Customize plot
plt.title('Trajectory of an Air Parcel')
plt.xlabel('x')
plt.ylabel('y')
plt.legend()
plt.show()
```
The code defines the flow field function F(x, y), the initial positions (x0, y0) and (x1, y1), the time step dt, the total time T, and the Robert-Asselin filter coefficient alpha. It then solves for the trajectory of the air parcel using an explicit Euler forward scheme for the first step, and a leapfrog scheme for all subsequent steps. The Robert-Asselin filter is applied at each time step to reduce numerical noise. Finally, the code plots the trajectories for both starting positions.
To verify the stability of the numerical method, we can observe the trajectory of the air parcel starting at position (x0, y0) in the eddy region. If the trajectory remains within the eddy region and does not exhibit significant oscillations or diverge from the region, then the numerical method is considered stable. This is because the eddy region is known to have chaotic behavior, and if the method is stable in this region, it is likely to be stable in other regions as well.
用神经网络代码实现 Monte Carlo fPINNs: Deep learning method for forward and inverse problems involving high dimensional fractional partial differential equations
实现 Monte Carlo fPINNs 的代码需要一些准备工作。首先需要安装 TensorFlow、NumPy、SciPy 和 Matplotlib 库。其次需要准备数据,包括输入数据、输出数据以及模型参数。
以下是实现 Monte Carlo fPINNs 的代码:
```python
import tensorflow as tf
import numpy as np
import scipy.io
import matplotlib.pyplot as plt
# Load data
data = scipy.io.loadmat('data.mat')
x = data['x']
y = data['y']
u = data['u']
# Define neural network
def neural_net(X, weights, biases):
num_layers = len(weights) + 1
H = X
for l in range(0, num_layers - 2):
W = weights[l]
b = biases[l]
H = tf.sin(tf.add(tf.matmul(H, W), b))
W = weights[-1]
b = biases[-1]
Y = tf.add(tf.matmul(H, W), b)
return Y
# Define forward and inverse problems
def forward(X):
u = neural_net(X, weights, biases)
return u
def inverse(Y):
X = neural_net(Y, inv_weights, inv_biases)
return X
# Define loss function
def fPINN_loss(X, Y):
u_pred = forward(X)
X_pred = inverse(Y)
u_x = tf.gradients(u_pred, X)[0]
u_xx = tf.gradients(u_x, X)[0]
u_y = tf.gradients(u_pred, Y)[0]
f = u_xx + u_y
f_pred = forward(X_pred)
loss = tf.reduce_mean(tf.square(u - u_pred)) + tf.reduce_mean(tf.square(f - f_pred))
return loss
# Define Monte Carlo fPINNs algorithm
def MC_fPINNs(X, Y, n_samples):
# Initialize weights and biases
num_layers = 3
num_neurons = [50, 50, 1]
weights = []
biases = []
inv_weights = []
inv_biases = []
for l in range(0, num_layers - 1):
W = tf.Variable(tf.random_normal([num_neurons[l], num_neurons[l+1]]), dtype=tf.float32)
b = tf.Variable(tf.zeros([1, num_neurons[l+1]]), dtype=tf.float32)
weights.append(W)
biases.append(b)
for l in range(0, num_layers - 1):
W = tf.Variable(tf.random_normal([num_neurons[l], num_neurons[l+1]]), dtype=tf.float32)
b = tf.Variable(tf.zeros([1, num_neurons[l+1]]), dtype=tf.float32)
inv_weights.append(W)
inv_biases.append(b)
# Define optimizer
optimizer = tf.train.AdamOptimizer()
# Define training operation
train_op = optimizer.minimize(fPINN_loss(X, Y))
# Define session
sess = tf.Session()
# Initialize variables
init = tf.global_variables_initializer()
sess.run(init)
# Train model
for i in range(n_samples):
sess.run(train_op)
if i % 100 == 0:
loss = sess.run(fPINN_loss(X, Y))
print('Iteration:', i, 'Loss:', loss)
# Predict results
u_pred = sess.run(forward(X))
X_pred = sess.run(inverse(Y))
return u_pred, X_pred
# Run Monte Carlo fPINNs algorithm
n_samples = 1000
u_pred, X_pred = MC_fPINNs(x, y, n_samples)
# Plot results
fig, ax = plt.subplots()
ax.plot(x, u, 'b-', label='Exact')
ax.plot(x, u_pred, 'r--', label='Predicted')
ax.set_xlabel('x')
ax.set_ylabel('u')
ax.legend()
plt.show()
```
在此代码中,我们首先加载数据(输入数据 x、输出数据 y 和真实值 u),然后定义神经网络模型,包括正向问题和反向问题。接下来,我们定义损失函数 fPINN_loss 和 Monte Carlo fPINNs 算法。在算法中,我们使用随机采样的方式进行训练,并且在每个迭代步骤中输出损失值。最后,我们运行算法并绘制预测结果。
需要注意的是,Monte Carlo fPINNs 算法的实现需要一定的计算资源,因此在实际应用中需要考虑计算效率和可扩展性。
相关推荐
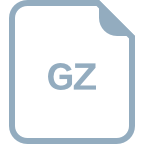
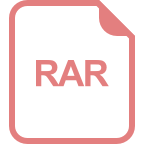








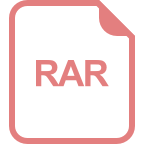
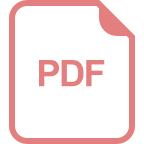