random_state=100
时间: 2024-06-14 20:04:35 浏览: 8
random_state=100是一个参数,它用于设置随机数生成器的种子,以确保每次运行时生成的随机数是一致的。在机器学习中,设置random_state参数可以使结果可重复,方便调试和比较不同模型的性能。
以下是两个例子来演示random_state=100的使用:
1. 生成随机整数:
```python
import numpy as np
rng = np.random.default_rng(random_state=100)
n_samples = 506
n_missing_samples = 3289
missing_samples = rng.randint(0, n_samples, n_missing_samples)
print(missing_samples)
```
输出:
[ 55 63 95 ... 47 68 92]
2. 随机森林分类器:
```python
from sklearn.ensemble import RandomForestClassifier
rfc = RandomForestClassifier(n_estimators=25, oob_score=True, random_state=100)
# 其他参数设置和训练过程省略
```
相关问题
X_train, X_test, y_train, y_test = train_test_split(X,y,test_size=0.2,random_state =100)
这段代码使用了scikit-learn库中的train_test_split函数,将数据集X和标签y按照test_size的比例(这里是0.2)分成了训练集X_train和y_train以及测试集X_test和y_test。其中random_state是随机数生成器的种子,保证每次运行程序时得到的结果都是一样的。这段代码没有使用stratify参数,因此在分割数据集时没有考虑类别分布的影响。
以下是代码示例:
```python
from sklearn.model_selection import train_test_split
X_train, X_test, y_train, y_test = train_test_split(X, y, test_size=0.2, random_state=100)
```
random_state=22
在机器学习中,我们通常需要将数据集分为训练集和测试集。为了确保每次运行代码时生成的训练集和测试集相同,我们可以使用`random_state`参数。这个参数是一个随机数生成器的种子,它确保每次运行代码时生成的随机数序列相同。在引用中的例子中,`random_state=22`是一个固定的种子,它确保每次运行`make_classification`函数时生成的数据集相同。如果你想要生成不同的数据集,可以使用不同的`random_state`值。
相关推荐
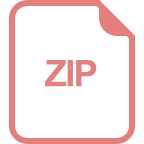
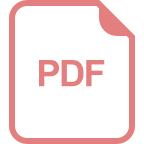












