temp = np.array(data["close"]) t = adfuller(temp) # ADF检验 output=pd.DataFrame(index=['Test Statistic Value', "p-value", "Lags Used", "Number of Observations Used","Critical Value(1%)","Critical Value(5%)","Critical Value(10%)"],columns=['value']) output['value']['Test Statistic Value'] = t[0] output['value']['p-value'] = t[1] output['value']['Lags Used'] = t[2] output['value']['Number of Observations Used'] = t[3] output['value']['Critical Value(1%)'] = t[4]['1%'] output['value']['Critical Value(5%)'] = t[4]['5%'] output['value']['Critical Value(10%)'] = t[4]['10%'] print(output) print(acorr_ljungbox(data["close"], lags=1)) data1= data["close"].diff(1) plt.figure(figsize=(10, 6)) data1.plot() plt.xlabel('日期',fontsize=12,verticalalignment='top') plt.ylabel('差分',fontsize=14,horizontalalignment='center') plt.show() temp = np.diff(data["close"]) t = adfuller(temp) # ADF检验 output=pd.DataFrame(index=['Test Statistic Value', "p-value", "Lags Used", "Number of Observations Used","Critical Value(1%)","Critical Value(5%)","Critical Value(10%)"],columns=['value']) output['value']['Test Statistic Value'] = t[0] output['value']['p-value'] = t[1] output['value']['Lags Used'] = t[2] output['value']['Number of Observations Used'] = t[3] output['value']['Critical Value(1%)'] = t[4]['1%'] output['value']['Critical Value(5%)'] = t[4]['5%'] output['value']['Critical Value(10%)'] = t[4]['10%'] print(output) exit()
时间: 2023-10-08 07:08:49 浏览: 40
这段代码首先对数据进行ADF检验,判断时间序列数据的平稳性。然后,它计算并输出了ADF检验的结果,包括测试统计值、p值、使用的滞后阶数、使用的观测值数量以及临界值(1%、5%、10%)。
接下来,代码对数据进行了一阶差分,并绘制了差分后的时间序列图。
之后,再次对差分后的数据进行ADF检验,并输出了结果。
最后,代码使用exit()函数退出程序。
相关问题
但是我已经声明过了 class satDataSet(data.Dataset): def __init__(self,root,filetypefile,transform=None): super(satDataSet,self).__init__() self.root = root self.files = [] self.transforms = transform df = pd.read_csv(filetypefile,names=['filename','satType']) for adf in df.values: dat_file = os.path.join(self.root,r"%s" % adf[0]) typename = int("%d" % adf[1]) self.files.append({ "datFile":dat_file, "typename":typename}) def __len__(self): return len(self.files) # 返回数据集的长度 def __getitem__(self, index): ##一张图像一张图像的读取,并处理,即三维的 datafiles = self.files[index] label = datafiles["typename"] #satdata = torch.from_numpy(np.load(datafiles["datFile"])["sat"]) satdata = np.load(datafiles["datFile"])["sat"] satdata = np.transpose(satdata, (1, 2, 0)) #print("satdata.shape",satdata.shape) ## 将数据纬度转化成(100, 101, 19)将通道数放在最后 # 将 NumPy 数组转换为 PIL 图像对象 #satdata = Image.fromarray(satdata) satdata = preprocess_data(satdata,opt) satdata = torch.from_numpy(satdata.transpose((2, 0, 1))) # 转换为 PyTorch 张量 label = torch.from_numpy(np.array([label])) return satdata,label
根据你提供的代码,你定义了一个名为satDataSet` 的类,该类继承自 `data.Dataset`。这是一个自定义的数据集类,用于加载和处理卫星图像数据集。
在这个自定义数据集类中,你实现了 `__init__`、`__len__` 和 `__getitem__` 方法。其中,`__init__` 方法初始化了数据集的根目录、文件列表和转换函数。`__len__` 方法返回了数据集的长度,即文件列表的长度。`__getitem__` 方法根据给定的索引,从文件列表中获取对应的文件名、标签,并进行相应的数据处理和转换操作。
这样,通过实现这些方法,你可以使用这个自定义数据集类来创建数据加载器,并在训练过程中迭代地获取样本数据。
请注意,这个自定义数据集类并不是 Python 的内置类或函数,而是你根据 `data.Dataset` 类进行的自定义实现。在使用时需要确保导入正确的模块,并按照正确的方式调用和使用该类。
Traceback (most recent call last): File "ADF.py", line 18, in <module> atoms.set_calculator(SinglePointCalculator(atoms, energy=0, forces=np.zeros((len(atoms), 3)), stress=np.zeros(6), neighborlist=nl)) File "/export/home/anliying/.local/lib/python3.8/site-packages/ase-3.22.1-py3.8.egg/ase/calculators/singlepoint.py", line 22, in init assert property in all_properties AssertionError。import numpy as np from ase.io import read from ase.build import make_supercell from ase.visualize import view from ase.neighborlist import NeighborList from ase.calculators.singlepoint import SinglePointCalculator # 读入三个POSCAR文件,计算原子分布函数 structures = [] for file in ['structure1.cif', 'structure2.cif', 'structure3.cif']: atoms = read(file) # 构造超胞,避免周期性边界对ADF计算的影响 atoms = make_supercell(atoms, [[2, 0, 0], [0, 2, 0], [0, 0, 2]]) # 计算邻居列表 nl = NeighborList([1.2] * len(atoms), self_interaction=False) nl.update(atoms) # 将邻居列表传递给SinglePointCalculator atoms.set_calculator(SinglePointCalculator(atoms, energy=0, forces=np.zeros((len(atoms), 3)), stress=np.zeros(6), neighborlist=nl)) # 计算原子分布函数 adf = atoms.get_atomic_distribution_function() structures.append(adf) # 将ADF转化为特征矩阵 bins = np.linspace(0, 10, num=100) # 分100个bin adf_hists = [np.histogram(adf, bins=bins)[0] for adf in structures] feature_matrix = np.array(adf_hists) / [len(atoms) for atoms in structures] # 归一化特征矩阵 feature_matrix = feature_matrix / np.linalg.norm(feature_matrix, axis=1, keepdims=True),基于错误改代码
It seems that the error occurs because the `property` argument is not recognized by the `SinglePointCalculator` constructor.
To fix this error, you can replace the `property` argument with `properties` in the `SinglePointCalculator` constructor. The corrected code should look like this:
```
atoms.set_calculator(SinglePointCalculator(atoms, energy=0, forces=np.zeros((len(atoms), 3)), stress=np.zeros(6), properties=['energy', 'forces'], neighborlist=nl))
```
Note that I have also added the `properties` argument with the values `['energy', 'forces']` to specify the properties that the calculator should compute.
阅读全文
相关推荐
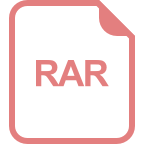
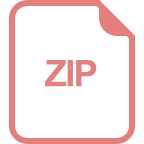











