if not os.path.exists(model_path): os.makedirs(model_path) joblib.dump(clf, model_path + 'model') clf = joblib.load(model_path+'model') print("训练之后的模型存放在model文件夹中")是正确保存了模型格式吗
时间: 2024-04-06 14:29:40 浏览: 18
是的,这段代码会检查模型路径是否存在,如果不存在则创建该路径,然后使用joblib.dump()方法将训练好的模型保存到该路径下。接着,使用joblib.load()方法从指定路径加载模型。最后,输出一条提示信息,说明训练好的模型存放在指定路径下的model文件夹中。
这样做可以确保模型被正确保存和加载,以便在需要的时候使用。但是需要注意的是,如果模型的超参数或其他相关信息发生了变化,可能需要重新训练模型。
相关问题
if not os.path.exists(LOG_PATH):
如果你想检查 `LOG_PATH` 路径是否存在并执行相应的操作,你可以使用以下代码:
```python
import os
if not os.path.exists(LOG_PATH):
# 执行你想要的操作,例如创建文件夹或者进行其他处理
os.makedirs(LOG_PATH)
```
在这个例子中,`os.path.exists(LOG_PATH)` 用于检查路径是否存在。如果路径不存在,`os.makedirs(LOG_PATH)` 可以用来创建该路径。你可以在这个条件下执行你想要的操作。
if not os.path.exists(self.model_save_dir): os.makedirs(self.model_save_dir)
这段代码应该也是在某个类的初始化方法中出现的。它的作用是检查模型保存目录是否存在,如果不存在则创建该目录。具体含义如下:
- `self.model_save_dir`:表示模型保存目录的路径,应该是该类的一个属性。
- `os.path.exists(self.model_save_dir)`:判断模型保存目录是否存在。
- `os.makedirs(self.model_save_dir)`:如果模型保存目录不存在,则创建该目录。
相关推荐
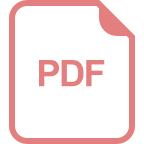
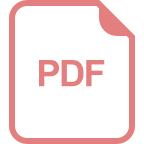
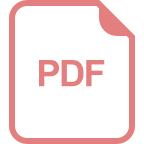












