能不能不用RandomForestClassifier类,用底层代码搭建一个随机森林的算法
时间: 2024-04-11 15:27:00 浏览: 56
当然可以!如果你想要自己搭建随机森林算法,可以使用决策树作为基础模型,并通过集成多个决策树来构建随机森林。下面是一个简单的示例代码:
```python
import numpy as np
# 定义一个决策树类
class DecisionTree:
def __init__(self):
self.tree = None
def fit(self, X, y):
# 在这里编写决策树的训练代码
# ...
def predict(self, X):
# 在这里编写决策树的预测代码
# ...
return y_pred
# 定义随机森林类
class RandomForest:
def __init__(self, n_estimators=100):
self.n_estimators = n_estimators
self.trees = []
def fit(self, X, y):
for _ in range(self.n_estimators):
# 随机采样训练数据
sample_indices = np.random.choice(len(X), size=len(X), replace=True)
X_sample = X[sample_indices]
y_sample = y[sample_indices]
# 创建并训练决策树
tree = DecisionTree()
tree.fit(X_sample, y_sample)
# 将训练好的决策树添加到随机森林中
self.trees.append(tree)
def predict(self, X):
# 对每个决策树进行预测,并进行投票
predictions = np.array([tree.predict(X) for tree in self.trees])
y_pred = np.mean(predictions, axis=0)
return y_pred
# 准备训练数据和目标变量
X_train = ...
y_train = ...
# 创建随机森林分类器
rf_classifier = RandomForest(n_estimators=100)
# 训练模型
rf_classifier.fit(X_train, y_train)
# 使用模型进行预测
X_test = ...
y_pred = rf_classifier.predict(X_test)
```
在上面的示例中,我们首先定义了一个`DecisionTree`类来实现决策树的训练和预测功能。然后,定义了一个`RandomForest`类来实现随机森林的训练和预测功能。在随机森林的训练过程中,我们通过随机采样训练数据来构建多个决策树,并将它们添加到随机森林中。最后,使用随机森林对测试数据进行预测。
当然,这只是一个简单的示例,实际应用中可能还需要考虑特征选择、调整模型参数、处理缺失值等步骤。希望对你有所帮助!如果有其他问题,请随时提问。
阅读全文
相关推荐
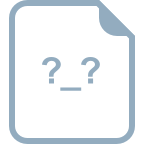
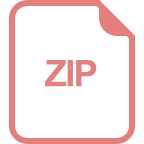
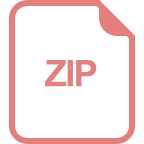















