Spark Structured Streaming使用的人多吗
时间: 2024-06-02 18:12:55 浏览: 120
Spark Structured Streaming已成为Apache Spark的一个重要组件,自2016年发布以来,已经被广泛使用。它在大规模数据处理和实时数据处理中都具有很高的可扩展性和灵活性,因此受到了很多企业和组织的欢迎。尤其是在流媒体、物联网、金融、电信和广告等领域,Spark Structured Streaming得到了广泛应用。
相关问题
spark structured streaming
Spark Structured Streaming is a high-level API built on top of Apache Spark that provides support for continuous stream processing. It allows developers to write streaming applications using the same DataFrame and SQL APIs that are used for batch processing.
Structured Streaming is based on the concept of a continuous processing engine that can process data in real-time as it arrives, rather than traditional batch processing that runs on a fixed schedule. It provides several key features, including:
1. High-level APIs for easy development and deployment of streaming applications
2. Support for both batch and streaming data processing
3. Built-in support for fault-tolerance and recovery
4. Integration with Apache Kafka, HDFS, and other data sources
5. Support for windowed aggregations and joins
Spark Structured Streaming also provides a powerful engine for managing and optimizing streaming workloads. It automatically manages the parallelism and resource allocation for processing data streams, optimizing performance and scalability.
Spark Structured Streaming和Spark Streaming的区别
Spark Structured Streaming 是 Spark 2.0 引入的一种全新的流处理方式,它是建立在 Spark SQL 引擎之上的,可以将流数据看成是结构化数据,并以表格的形式进行处理。相比于 Spark Streaming,它具有以下几点不同之处:
1. 处理方式不同:Spark Streaming 是基于 DStream 的离散流处理,而 Spark Structured Streaming 直接将流数据看成是连续的表格数据,可以和静态的表格数据一样处理。
2. 数据处理粒度不同:Spark Streaming 的数据处理粒度是每个微批次(batch)的数据,而 Spark Structured Streaming 的数据处理粒度是每条数据。
3. 数据处理模式不同:Spark Streaming 的处理模式是批处理,而 Spark Structured Streaming 则是以流的方式进行处理。
4. 数据处理延迟不同:Spark Streaming 的延迟一般在几秒到几十秒之间,而 Spark Structured Streaming 的延迟可以做到毫秒级别。
综上所述,Spark Structured Streaming 的设计目标是在保证高吞吐量和低延迟的同时,提供结构化的数据处理方式,使得数据处理更为方便和灵活。而 Spark Streaming 主要适用于需要对实时数据进行批处理的场景。
阅读全文
相关推荐
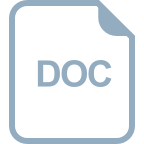
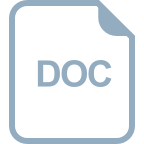
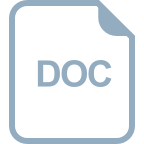
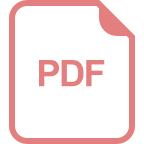





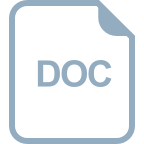
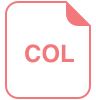


