用python基于ARIMA模型的股票数据完整代码
时间: 2024-03-24 17:40:02 浏览: 46
下面是一个完整的基于ARIMA模型分析股票数据的Python代码示例,其中使用了pandas和statsmodels库:
```
import pandas as pd
from statsmodels.tsa.arima_model import ARIMA
import matplotlib.pyplot as plt
# 导入股票数据
df = pd.read_csv('stock_data.csv')
df['Date'] = pd.to_datetime(df['Date'])
df.set_index('Date', inplace=True)
# 进行一阶差分,使时间序列平稳
df['Close_diff'] = df['Close'].diff()
df.dropna(inplace=True)
# 拟合ARIMA模型
model = ARIMA(df['Close'], order=(1, 1, 1))
results = model.fit()
print(results.summary())
# 可视化预测结果
fig, ax = plt.subplots()
ax.plot(df['Close'])
results.plot_predict('2021-01-01', '2022-01-01', ax=ax, plot_insample=False)
plt.show()
```
需要注意的是,这只是一个简单的示例代码,实际应用中可能需要进行更复杂的数据预处理和模型调参。同时,股票预测并不是一件容易的事情,需要结合更多的因素和指标考虑。
相关问题
用python基于ARIMA模型的股票数据预测
如果您已经按照上一条回答中的步骤,成功地拟合了ARIMA模型,那么可以使用拟合好的模型对未来的股票数据进行预测。
具体地,您可以按照以下步骤进行:
1. 使用拟合好的ARIMA模型对未来的数据进行预测。可以使用Python中的forecast方法进行预测。例如,如果您想预测未来5个时间点的股票价格,可以使用以下代码:
```python
# 使用拟合好的ARIMA模型进行预测
predict = model.forecast(steps=5)
```
这里的steps参数表示要预测的时间点个数,可以根据实际需要进行调整。
2. 将预测结果可视化。可以使用Python中的matplotlib库对预测结果进行可视化。例如,可以使用以下代码将预测结果绘制成折线图:
```python
# 将预测结果绘制成折线图
plt.plot(predict, color='blue')
plt.title('Stock Price Prediction')
plt.xlabel('Time')
plt.ylabel('Price')
plt.show()
```
这样就可以使用基于ARIMA模型的股票数据预测了。需要注意的是,预测结果仅供参考,实际情况可能会受到各种因素的影响而偏离预测结果。因此,在进行股票投资决策时,需要综合考虑多种因素,并且不要过度依赖单一的预测模型。
用Python写出基于ARIMA 模型的股票预测
ARIMA模型是一种基于时间序列的预测模型,可以用于股票价格的预测。在Python中,可以使用statsmodels库来实现ARIMA模型的构建和预测。以下是一个基于ARIMA模型的股票价格预测的示例代码:
```python
import pandas as pd
import numpy as np
import matplotlib.pyplot as plt
from statsmodels.tsa.arima_model import ARIMA
from sklearn.metrics import mean_squared_error
# Load dataset
df = pd.read_csv('stock_prices.csv')
# Split to train and test sets
train = df[:500]
test = df[500:]
# Build ARIMA model
model = ARIMA(train['price'], order=(5, 1, 0))
model_fit = model.fit(disp=0)
# Make predictions
predictions = model_fit.forecast(steps=len(test))[0]
# Evaluate model
mse = mean_squared_error(test['price'], predictions)
rmse = np.sqrt(mse)
print(f'RMSE: {rmse}')
# Plot results
plt.plot(train['price'], label='Train')
plt.plot(test['price'], label='Test')
plt.plot(test.index, predictions, label='Predictions')
plt.legend()
plt.show()
```
在这个示例中,我们首先加载了股票价格的数据集,并将其分割成训练和测试集。然后,我们使用ARIMA模型拟合训练数据,并用其预测测试数据。最后,我们使用均方根误差(RMSE)评估了模型的性能,并将训练、测试和预测结果可视化。
相关推荐
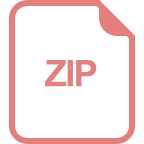
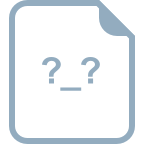
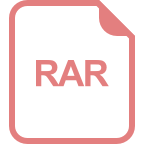












