为什么numpy.random.normal在每一次for循环中值一样
时间: 2023-12-17 20:05:24 浏览: 139
`numpy.random.normal`函数是用来产生服从正态分布的随机数的,它的参数包括平均值、标准差和样本数。如果你在每次循环中只传入相同的参数,那么它产生的随机数就会是一样的。解决这个问题的方法是在每次循环中使用不同的参数,例如可以使用时间戳来生成不同的种子,然后传入`numpy.random.seed`函数中,使得每次循环中生成的随机数序列都不同。示例代码如下:
```python
import numpy as np
import time
for i in range(10):
np.random.seed(int(time.time()))
x = np.random.normal(0, 1, 10)
print(x)
```
在上面的示例代码中,我们使用了当前时间的时间戳作为随机数种子,保证了每次循环中生成的随机数都是不同的。
相关问题
(2)生成代码,实现图像分别添加椒盐噪声与乘性噪声,手写实现3*3窗口均值滤波与中值滤波,并比 较两种方法在不同噪声下的去噪效果。提示:嵌套使用for循环语句
为了手写实现图像的椒盐噪声、乘性噪声的添加,以及3x3窗口的均值滤波和中值滤波,我们可以使用Python的PIL库处理图片,numpy处理数组运算。以下是部分代码示例:
```python
from PIL import Image
import numpy as np
# 加载图像
def load_image(file_path):
return Image.open(file_path).convert('L') # 黑白图像处理
# 添加椒盐噪声
def add_salt_pepper_noise(image, probability=0.05):
noisy = np.array(image)
salt_mask = np.random.rand(*noisy.shape) < probability
pepper_mask = np.invert(salt_mask)
noisy[salt_mask] = 255 # 将随机位置设为最大亮度
noisy[pepper_mask] = 0 # 将随机位置设为最小亮度
return Image.fromarray(noisy)
# 乘性噪声
def add_multiplicative_noise(image, factor=0.5):
noisy = np.array(image)
noisy *= np.random.normal(1, factor, size=noisy.shape) # 随机乘以一个小于1的因子
return Image.fromarray(np.uint8(noisy))
# 3x3窗口均值滤波
def mean_filter(image, kernel_size=3):
padded_img = np.pad(image, pad_width=(kernel_size // 2, kernel_size // 2), mode='edge')
filtered = np.zeros_like(padded_img)
for i in range(kernel_size, len(padded_img) - kernel_size + 1):
for j in range(kernel_size, len(padded_img[0]) - kernel_size + 1):
filtered[i, j] = np.mean(padded_img[i:i+kernel_size, j:j+kernel_size])
return filtered
# 中值滤波
def median_filter(image, kernel_size=3):
padded_img = np.pad(image, pad_width=(kernel_size // 2, kernel_size // 2), mode='edge')
filtered = np.zeros_like(padded_img)
for i in range(kernel_size, len(padded_img) - kernel_size + 1):
for j in range(kernel_size, len(padded_img[0]) - kernel_size + 1):
filtered[i, j] = np.median(padded_img[i:i+kernel_size, j:j+kernel_size])
return filtered
# 使用以上函数对原始图像进行操作并比较结果
original_image = load_image("input.jpg")
salt_and_pepper_noisy = add_salt_pepper_noise(original_image)
multiplicative_noisy = add_multiplicative_noise(original_image)
filtered_mean = mean_filter(salt_and_pepper_noisy)
filtered_median = median_filter(salt_and_pepper_noisy)
# 可能需要将过滤后的图像保存或显示出来,以便对比
mean_filtered_image = Image.fromarray(filtered_mean)
median_filtered_image = Image.fromarray(filtered_median)
# 比较结果
# ...
中值滤波数据降噪 python
中值滤波是一种非线性数字滤波方法,可以有效地降低信号的噪声。在 Python 中,可以使用 NumPy 库中的 median 函数来实现中值滤波。
以下是一个简单的示例代码,演示如何使用中值滤波对数据进行降噪:
```python
import numpy as np
# 生成含噪声的数据
data = np.random.normal(0, 0.5, 1000)
# 添加噪声
noise = np.random.normal(0, 0.2, 1000)
data = data + noise
# 中值滤波
filtered_data = np.zeros_like(data)
window_size = 5
for i in range(len(data)):
if i < window_size//2 or i >= len(data)-window_size//2:
filtered_data[i] = data[i]
else:
filtered_data[i] = np.median(data[i-window_size//2:i+window_size//2+1])
# 绘制结果
import matplotlib.pyplot as plt
fig, ax = plt.subplots()
ax.plot(data, label='Original Data')
ax.plot(filtered_data, label='Filtered Data')
ax.legend()
plt.show()
```
在上述代码中,首先生成了一个含噪声的数据集。然后,使用中值滤波对数据进行降噪,设置窗口大小为 5。最后,使用 Matplotlib 库绘制了原始数据和降噪后的数据的图表。
阅读全文
相关推荐
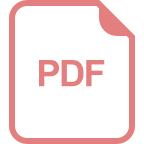
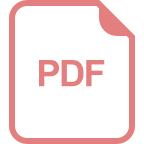
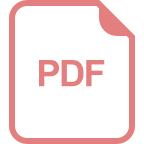
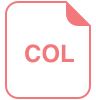
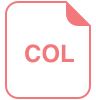
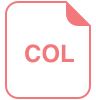
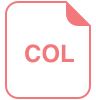
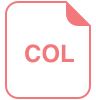
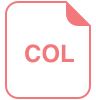
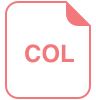






