with tf.device("/cpu:0"): 改为gpu训练
时间: 2024-03-05 15:50:10 浏览: 16
您可以将 `tf.device("/cpu:0")` 修改为 `tf.device("/gpu:0")`,其中的数字 0 表示使用第一个 GPU 设备。如果您有多个 GPU 设备,可以选择使用其他的设备,例如 `/gpu:1`、`/gpu:2` 等。修改后的代码如下所示:
```python
with tf.device("/gpu:0"):
# 在这里添加您的 TensorFlow 训练代码
```
这样您的 TensorFlow 训练代码就会在 GPU 上运行。请注意,为了在 GPU 上运行 TensorFlow,您需要安装 GPU 版本的 TensorFlow 并且有可用的 GPU 设备。
相关问题
if device_type == Devices.cpu: with tf.device('/CPU:0'): yield'
这是一个使用 TensorFlow 框架的 Python 代码片段,它用于指定在 CPU 上运行代码。这段代码使用了 Python 的生成器函数(yield),它返回一个迭代器。当你调用这个生成器函数时,它会返回一个迭代器对象。当你在迭代器上调用 next() 方法时,它会执行这段代码,直到遇到 yield 关键字,然后将 yield 后面的值返回给调用者。在这里,这段代码返回字符串 ' ',但实际上这个代码片段应该返回更多的代码,因为这个函数是一个生成器函数。
InvalidArgumentError (see above for traceback): Cannot assign a device for operation 'IteratorToStringHandle': Operation was explicitly assigned to /device:GPU:0 but available devices are [ /job:localhost/replica:0/task:0/device:CPU:0 ]. Make sure the device specification refers to a valid device. [[{{node IteratorToStringHandle}} = IteratorToStringHandle[_device="/device:GPU:0"](IteratorV2)]]
这个错误是因为您尝试在 GPU 上执行一个不支持 GPU 的操作。具体地说,在您的代码中,`IteratorToStringHandle` 这个操作被指定在了 `/device:GPU:0` 上执行,但是这个操作只能在 CPU 上运行。因此,TensorFlow 抛出了这个错误。
要解决这个问题,您需要将 `IteratorToStringHandle` 这个操作从 GPU 设备上移除,使其能够在 CPU 上运行。一种简单的方法是将 `with tf.device("/gpu:0"):` 改为 `with tf.device("/cpu:0"):`,这样包括 `IteratorToStringHandle` 在内的所有操作都将在 CPU 上运行。修改后的代码如下所示:
```python
with tf.device("/cpu:0"):
# 在这里添加您的 TensorFlow 训练代码
```
如果您需要将某些操作固定在 GPU 上执行,可以使用 `tf.device` 和 `tf.compat.v1.disable_eager_execution()` 来手动控制 TensorFlow 的计算图。具体来说,您可以在 `with tf.device("/gpu:0"):` 块中创建一个 TensorFlow 计算图,并使用 `tf.compat.v1.Session` 来运行这个计算图。这样,您就可以将某些操作固定在 GPU 上运行,而将其他操作放在 CPU 上运行。下面是一个示例代码:
```python
import tensorflow as tf
# 关闭 Eager Execution
tf.compat.v1.disable_eager_execution()
# 定义一个 TensorFlow 计算图
with tf.device("/gpu:0"):
x = tf.constant([1.0, 2.0, 3.0])
y = tf.constant([4.0, 5.0, 6.0])
z = tf.add(x, y)
# 创建一个 TensorFlow 会话并运行计算图
with tf.compat.v1.Session() as sess:
# 将 z 固定在 GPU 上运行
result = sess.run(z)
print(result)
```
在这个示例代码中,`x` 和 `y` 这两个操作被固定在 GPU 上执行,而 `z` 这个操作则会被自动放到 GPU 上执行。最终,您将获得一个包含 `[5.0, 7.0, 9.0]` 的 NumPy 数组。
相关推荐
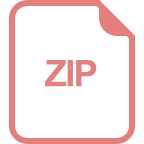
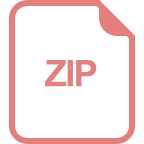












