python 声音克隆
时间: 2023-11-25 15:02:38 浏览: 86
Python声音克隆是指使用Python编程语言来实现声音的复制和克隆。在Python中,我们可以利用一些库和工具来处理声音文件,比如使用SciPy库来进行声音的读取和写入,使用NumPy库来进行声音信号的处理和分析,使用PyDub库来进行声音的剪切、合并和混音等操作。
通过Python编程,我们可以实现声音克隆的功能,比如可以将一个声音文件完整地复制一份,或者对声音文件进行一些变换后再复制,从而实现声音的克隆效果。例如,我们可以利用Python来实现声音的重复播放、音调的变换、音量的调整等功能,从而实现声音的克隆。
另外,Python还可以结合一些机器学习和人工智能的技术,来实现更加高级的声音克隆功能。比如可以利用深度学习模型来实现语音的识别和合成,从而实现声音的生成和复制。这些技术可以应用在语音助手、语音合成等领域,从而实现更加智能和自然的声音克隆效果。
总之,通过Python编程可以实现各种各样的声音克隆功能,从简单的复制和变换到复杂的语音合成和识别,都可以通过Python来实现。这些技术可以应用在音频处理、智能语音系统等领域,为人们的生活和工作带来便利。
相关问题
python声音克隆
声音克隆是指使用计算机技术来模拟和复制人类的声音。在Python中,有一个名为Real-Time-Voice-Cloning的项目可以实现声音克隆。该项目提供了代码和预训练的模型,可以用于合成音频和模拟特定人物的声音。要使用Real-Time-Voice-Cloning项目,需要安装一些必要的库,如umap-learn、visdom、librosa、matplotlib、numpy、scipy、tqdm、sounddevice、SoundFile、Unidecode、inflect、PyQt5、multiprocess、numba和webrtcvad。具体的安装步骤可以参考Real-Time-Voice-Cloning项目的文档。[1][2][3]
python的克隆技术
在Python中,克隆(Clone)技术通常指的是创建对象的副本,使得新创建的对象与原对象具有相同的属性和状态。这在数据结构、对象复用以及避免副作用等方面很有用。Python提供了几种方式来实现对象的克隆:
1. **浅复制(Shallow Copy)**: 使用`copy`模块的`copy()`或`deepcopy()`函数,如果对象是简单类型(如数字、字符串)或者引用的对象内部数据结构是不可变的(如元组),浅复制会创建一个引用。对于可变容器类型(如列表、字典),浅复制会复制指向内容的引用,而不是内容本身。
- `copy.copy()`:创建浅复制。
- `copy.deepcopy()`:创建深复制,即使对象内部有循环引用也能正确处理。
2. **深复制(Deep Copy)**: 如上述的`deepcopy()`函数,它会递归地复制嵌套的数据结构,确保每个子对象也被独立复制,不共享内存。
3. **自定义克隆**:如果你有一个自定义对象,没有使用内置库提供的方法,你可以重写`__copy__()`和`__deepcopy__()`方法来自定义复制行为。
- `__copy__()`: 如果实现了这个方法,当使用浅复制时会被调用。
- `__deepcopy__()`: 当使用深复制时会被调用。
4. **使用序列解包和生成器表达式**:对于简单数据结构,可以通过序列解包和生成器表达式进行快速克隆。
相关推荐
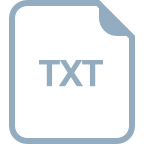












