def get_completion_from_messages(messages, model="gpt-3.5-turbo", temperature=0): response = openai.ChatCompletion.create( model=model, messages=messages, temperature=temperature, ) print(str(response.choices[0].message)) return response.choices[0].messag和def get_completion_from_messages(messages, model="gpt-3.5-turbo", temperature=0): response = openai.ChatCompletion.create( model=model, messages=messages, temperature=temperature, ) print(str(response.choices[0].message)) return response.choices[0].messag有什么不同
时间: 2023-06-19 09:04:11 浏览: 63
这两个函数的代码实现是相同的,都是使用 OpenAI 的 API 进行对话生成。但是它们的返回值不同:
- 第一个函数返回的是 `response.choices[0].message`,即生成的回复内容。
- 第二个函数返回的是 `response.choices[0].text`,应该是笔误,应该是 `response.choices[0].message`。
因此,这两个函数的主要区别在于返回值的不同。
相关问题
def get_completion(prompt, model="gpt-3.5-turbo"): messages = [{"role": "user", "content": prompt}] response = openai.ChatCompletion.create( model=model, messages=messages, temperature=0, # this is the degree of randomness of the model's output ) return response.choices[0].message["content"] 和 def get_completion_from_messages(messages, model="gpt-3.5-turbo", temperature=0): response = openai.ChatCompletion.create( model=model, messages=messages, temperature=temperature, # this is the degree of randomness of the model's output ) # print(str(response.choices[0].message)) return response.choices[0].message["content"] 有什么不同
这两个函数的主要区别在于输入参数。第一个函数需要一个字符串作为对话的开端,然后使用指定的模型生成对话的下一步。而第二个函数需要一个包含对话历史记录的列表,然后使用指定的模型生成对话的下一步。第二个函数更加灵活,因为它允许在对话中添加更多的历史信息,以便模型生成更加准确的回复。此外,第二个函数还可以指定温度参数,以控制模型生成回复时的随机性。
def get_completion(prompt, model="gpt-3.5-turbo"): messages = [{"role": "user", "content": prompt}] response = openai.ChatCompletion.create( model=model, messages=messages, temperature=0, return response.choices[0].message["content"]是什么意思
这是一个 Python 函数,用于使用 OpenAI 的 ChatCompletion API 进行对话生成。该函数的输入参数包括 prompt(即用户在对话中输入的内容)和 model(即用于生成对话的模型)。函数内部创建了一个包含用户输入内容的消息列表,然后调用 OpenAI 的 API 生成对话,并从 API 返回的响应中提取出生成的回复内容,最后将其作为函数的输出结果返回。其中 temperature 参数用于控制生成回复的随机程度,这里设置为 0 表示生成的回复尽可能符合模型预测的结果。
相关推荐
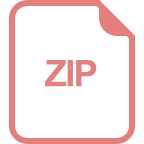
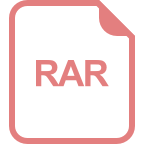












