解释这段代码def cluster_function(data, para_cluster=np.arange(1, 20, 2)): score = [] optimal_score = 0 for n in para_cluster: estimator = KMeans(init='k-means++', n_clusters=n) model = estimator.fit(data) s = calinski_harabasz_score(data, model.predict(data)) score.append(s) if s > optimal_score: optimal_score = s optimal_estimator=model plt.figure(figsize=(10, 10)) plt.plot(para_cluster, score, 'bo-') plt.xlabel('k-num') plt.ylabel('scores') plt.show() optimal_n_id = np.argmax(score) optimal_n = para_cluster[optimal_n_id] optimal_score = score[optimal_n_id] print('最终数据被分为', optimal_n, '簇') print('CH系数为:', optimal_score) return optimal_estimator , optimal_n # 保存模型 data = XXXX #这里的data是只有n行*96列的数据 estimator, n_cluster = cluster_function(data,para_cluster=np.arange(3,30,1)) joblib.dump(estimator, './xxx.pki')
时间: 2024-04-04 11:32:20 浏览: 104
这段代码实现了一个 KMeans 聚类算法,并且进行了 CH 系数的评估。具体实现如下:
1. 输入数据为 data,其中数据有 n 行和 96 列。
2. para_cluster 表示聚类的数量,从 1 到 19,步长为 2。
3. 遍历 para_cluster,对每个聚类数量 n,使用 KMeans 算法对数据进行聚类,得到一个模型 estimator。
4. 使用 calinski_harabasz_score 函数计算 CH 系数,将其存储在 score 列表中。
5. 在每次遍历时,判断当前聚类模型的 CH 系数是否大于最优 CH 系数,如果是,则更新最优 CH 系数和最优模型 estimator。
6. 使用 matplotlib 库绘制聚类数量与 CH 系数之间的关系曲线。
7. 找到最优的聚类数量和对应的 CH 系数,并输出结果。
8. 最后将最优模型 estimator 保存到本地。
总体来说,这段代码实现了一个聚类分析的过程,并且通过 CH 系数评估了聚类效果,得到最优的聚类数量和对应的聚类模型。
相关问题
def map_data(data_map, axis_num): data_map = np.max(data_map, axis=axis_num) data_map -= data_map.min() data_map = data_map / data_map.max() data_map = np.array(data_map * 255, dtype=np.uint8) return data_map 翻译这段代码
这段代码的作用是将输入的二维数组或多维数组的每个元素映射到0到255之间的整数,并将其作为输出返回。
具体实现步骤如下:
1. 第一个参数data_map是一个二维或多维数组,表示待映射的数据。
2. 第二个参数axis_num表示在哪个轴上求最大值,比如axis_num=0表示在第0维上求最大值,返回一个由每列最大值组成的一维数组。axis_num=1表示在第1维上求最大值,返回一个由每行最大值组成的一维数组。
3. 对于返回的一维数组,我们用np.max函数求得最大值,然后用这个最大值来对原数组进行归一化,即data_map = data_map / data_map.max()。
4. 将0~1之间的数值映射到0~255之间的整数,即data_map = np.array(data_map * 255, dtype=np.uint8)。
5. 返回映射后的数组data_map。
运行class GuidedBackpropReLUModel: def __init__(self, model, use_cuda): self.model = model self.model.eval() self.cuda = use_cuda if self.cuda: self.model = model.cuda() for idx, module in self.model.features._modules.items(): if module.__class__.__name__ == 'ReLU': self.model.features._modules[idx] = GuidedBackpropReLU() def forward(self, input): return self.model(input) def forward_static(self, input): if self.cuda: output = self.forward(input.cuda()) else: output = self.forward(input) return output def __call__(self, input, index = None): output = self.forward_static(input) if index == None: index = np.argmax(output.cpu().data.numpy()) one_hot = np.zeros((1, output.size()[-1]), dtype = np.float32) one_hot[0][index] = 1 one_hot = Variable(torch.from_numpy(one_hot), requires_grad = True) if self.cuda: one_hot = torch.sum(one_hot.cuda() * output) else: one_hot = torch.sum(one_hot * output) one_hot.backward() output = input.grad.cpu().data.numpy() output = output[0,:,:,:] return output报错Legacy autograd function with non-static forward method is deprecated. Please use new-style autograd function with static forward method. 如何修改代码
这个错误提示是因为代码中使用了旧版本的autograd函数,需要使用新版本的autograd函数。可以使用`@staticmethod`修饰`GuidedBackpropReLU`类中的`forward`方法,将其转换为静态方法。修改后的代码如下:
```
class GuidedBackpropReLU(nn.ReLU):
@staticmethod
def forward(input):
output = torch.clamp(input, min=0.0)
grad = torch.zeros_like(output)
grad[output > 0] = 1.0
output = output * grad
return output
class GuidedBackpropReLUModel:
def __init__(self, model, use_cuda):
self.model = model
self.model.eval()
self.cuda = use_cuda
if self.cuda:
self.model = model.cuda()
for idx, module in self.model.features._modules.items():
if module.__class__.__name__ == 'ReLU':
self.model.features._modules[idx] = GuidedBackpropReLU()
def forward(self, input):
return self.model(input)
def forward_static(self, input):
if self.cuda:
output = self.forward(input.cuda())
else:
output = self.forward(input)
return output
def __call__(self, input, index=None):
output = self.forward_static(input)
if index is None:
index = np.argmax(output.cpu().data.numpy())
one_hot = np.zeros((1, output.size()[-1]), dtype=np.float32)
one_hot[0][index] = 1
one_hot = Variable(torch.from_numpy(one_hot), requires_grad=True)
if self.cuda:
one_hot = torch.sum(one_hot.cuda() * output)
else:
one_hot = torch.sum(one_hot * output)
one_hot.backward()
output = input.grad.cpu().data.numpy()
output = output[0, :, :, :]
return output
```
阅读全文
相关推荐
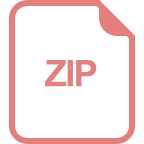
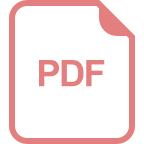














