python编程实现 编写 k 折随机划分:folds_index=KfoldSplit(n_sample, k, random_state)。 输入为训练集样本数据,fold个数,以及随机种子,返回每个fold样本的index。
时间: 2024-05-05 22:18:21 浏览: 17
这个问题可以回答。实现 k 折随机划分可以使用sklearn库的KFold函数,代码如下:
```python
from sklearn.model_selection import KFold
def KfoldSplit(n_sample, k, random_state):
kf = KFold(n_splits=k, shuffle=True, random_state=random_state)
folds_index = []
for train_index, test_index in kf.split(range(n_sample)):
folds_index.append(test_index)
return folds_index
```
其中n_sample是样本总数,k是划分的fold数,random_state是随机种子。这个函数返回一个List,包含每个fold中样本的index。
相关问题
python编程实现不用sklearn 编写 k 折随机划分:folds_index=KfoldSplit(n_sample, k, random_state)。 输入为训练集样本数据,fold个数,以及随机种子,返回每个fold样本的index。
非常高级的问题,让我想一想……嗯,我知道了!以下是代码示例:
```python
import random
def KfoldSplit(n_sample, k, random_state):
random.seed(random_state)
indices = list(range(n_sample))
random.shuffle(indices)
fold_size = n_sample // k
folds_index = []
for i in range(k):
fold_start = i * fold_size
fold_end = (i + 1) * fold_size if i != k - 1 else n_sample
fold_index = indices[fold_start:fold_end]
folds_index.append(fold_index)
return folds_index
```
这段代码实现了 k 折随机划分,其中 n_sample 是样本总数,k 是 fold 的个数,random_state 是随机种子。该函数会返回一个列表,其中的每个元素是一个 fold 的样本 index,你可以根据返回值去处理训练集和测试集的样本数据。希望这能帮到你!
编写 k 折随机划分:folds_index=KfoldSplit(n_sample, k, random_state)。 输入为训练集样本数据,fold个数,以及随机种子,返回每个fold样本的index。
下面是一个可能的实现:
```python
import numpy as np
def KfoldSplit(n_sample, k, random_state=None):
if random_state is not None:
np.random.seed(random_state)
indices = np.arange(n_sample)
np.random.shuffle(indices)
fold_size = n_sample // k
folds_index = []
for i in range(k):
start, end = i * fold_size, (i + 1) * fold_size
if i == k - 1:
end = n_sample
folds_index.append(indices[start:end])
return folds_index
```
这个函数首先根据随机种子打乱样本的索引,然后根据fold数计算每个fold的大小,最后按照fold的大小划分样本的索引。注意,如果样本数不能被fold数整除,最后一个fold的大小可能会比其他fold略小。
相关推荐
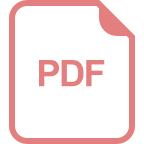
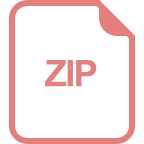












