import torch import torch.nn as nn from torch.nn import functional as F class RestNetBasicBlock(nn.Module): def __init__(self, in_channels, out_channels, stride): super(RestNetBasicBlock, self).__init__() self.conv1 = nn.Conv2d(in_channels, out_channels, kernel_size=3, stride=stride, padding=1) self.bn1 = nn.BatchNorm2d(out_channels) self.conv2 = nn.Conv2d(out_channels, out_channels, kernel_size=3, stride=stride, padding=1) self.bn2 = nn.BatchNorm2d(out_channels) def forward(self, x): output = self.conv1(x) output = F.relu(self.bn1(output)) output = self.conv2(output) output = self.bn2(output) return F.relu(x + output) ———————————————— 逐行解释以上代码
时间: 2024-04-17 14:25:03 浏览: 109
这段代码定义了一个名为`RestNetBasicBlock`的类,它是ResNet中的基本残差块。下面是对代码的逐行解释:
```python
import torch
import torch.nn as nn
from torch.nn import functional as F
```
首先导入了PyTorch库及其相关模块。
```python
class RestNetBasicBlock(nn.Module):
def __init__(self, in_channels, out_channels, stride):
super(RestNetBasicBlock, self).__init__()
self.conv1 = nn.Conv2d(in_channels, out_channels, kernel_size=3, stride=stride, padding=1)
self.bn1 = nn.BatchNorm2d(out_channels)
self.conv2 = nn.Conv2d(out_channels, out_channels, kernel_size=3, stride=stride, padding=1)
self.bn2 = nn.BatchNorm2d(out_channels)
```
接下来定义了一个名为`RestNetBasicBlock`的类,它继承自`nn.Module`类。构造函数`__init__`接受三个参数:`in_channels`表示输入特征图的通道数,`out_channels`表示输出特征图的通道数,`stride`表示卷积层的步长。
在构造函数中,定义了两个卷积层(`conv1`和`conv2`)和两个批归一化层(`bn1`和`bn2`)。这些层用于构建基本残差块。
```python
def forward(self, x):
output = self.conv1(x)
output = F.relu(self.bn1(output))
output = self.conv2(output)
output = self.bn2(output)
return F.relu(x + output)
```
`forward`方法定义了正向传播过程。给定输入`x`,首先通过第一个卷积层`conv1`进行卷积操作,得到`output`。然后将`output`通过批归一化层`bn1`和ReLU激活函数进行处理。
接下来,将处理后的特征图`output`再次通过第二个卷积层`conv2`进行卷积操作,得到最终的输出特征图。然后再将输出特征图通过批归一化层`bn2`进行处理。
最后,将输入特征图`x`与输出特征图相加,并通过ReLU激活函数进行处理,得到最终的输出。
这个基本残差块的设计遵循了ResNet的思想,通过跳跃连接将输入与输出相加,并使用ReLU激活函数来引入非线性。这样可以解决网络训练中的梯度消失问题,使得更深的网络能够更容易地训练和优化。
阅读全文
相关推荐
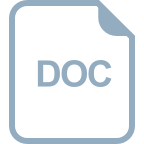
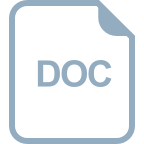
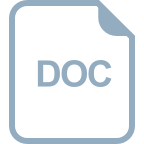
















