卷积神经网络实现识别手写数字mnist数据集运行代码
时间: 2023-07-25 15:03:30 浏览: 83
好的,以下是利用卷积神经网络实现手写数字识别的代码:
```python
import tensorflow as tf
from tensorflow.examples.tutorials.mnist import input_data
# 导入数据集
mnist = input_data.read_data_sets('MNIST_data', one_hot=True)
# 设置超参数
learning_rate = 0.001
epochs = 10
batch_size = 128
# 定义网络结构
x = tf.placeholder(tf.float32, [None, 784])
y = tf.placeholder(tf.float32, [None, 10])
x_image = tf.reshape(x, [-1,28,28,1])
conv1 = tf.layers.conv2d(x_image, 32, 5, activation=tf.nn.relu)
pool1 = tf.layers.max_pooling2d(conv1, 2, 2)
conv2 = tf.layers.conv2d(pool1, 64, 3, activation=tf.nn.relu)
pool2 = tf.layers.max_pooling2d(conv2, 2, 2)
fc1 = tf.contrib.layers.flatten(pool2)
fc1 = tf.layers.dense(fc1, 1024)
fc1 = tf.nn.relu(fc1)
fc1 = tf.layers.dropout(fc1, rate=0.5)
output = tf.layers.dense(fc1, 10)
# 定义损失函数和优化器
loss = tf.reduce_mean(tf.nn.softmax_cross_entropy_with_logits(logits=output, labels=y))
optimizer = tf.train.AdamOptimizer(learning_rate=learning_rate).minimize(loss)
# 定义评价指标
correct_prediction = tf.equal(tf.argmax(output, 1), tf.argmax(y, 1))
accuracy = tf.reduce_mean(tf.cast(correct_prediction, tf.float32))
# 训练模型
with tf.Session() as sess:
sess.run(tf.global_variables_initializer())
for epoch in range(epochs):
total_batch = mnist.train.num_examples // batch_size
for batch in range(total_batch):
batch_x, batch_y = mnist.train.next_batch(batch_size)
sess.run(optimizer, feed_dict={x: batch_x, y: batch_y})
# 每个epoch结束后计算在验证集上的准确率
valid_accuracy = sess.run(accuracy, feed_dict={x: mnist.validation.images, y: mnist.validation.labels})
print('Epoch {:<3} - Validation Accuracy: {}'.format(epoch, valid_accuracy))
# 在测试集上测试模型
test_accuracy = sess.run(accuracy, feed_dict={x: mnist.test.images, y: mnist.test.labels})
print('Test Accuracy: {}'.format(test_accuracy))
```
运行该代码可以得到手写数字识别模型在测试集上的准确率。
相关推荐
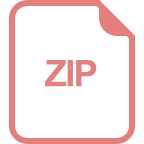
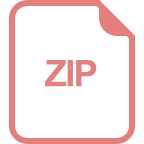
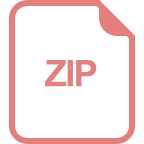
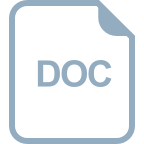
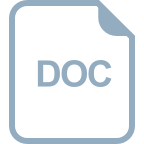
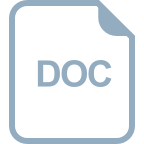
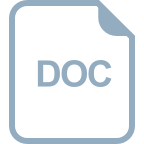
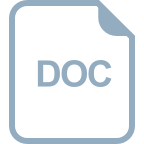


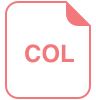






