df.to_excel("E:\python-file\lab3\Scores.xlsx", index=False)有什么问题
时间: 2023-10-08 10:11:16 浏览: 80
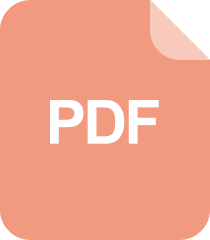
解决Python pandas df 写入excel 出现的问题
这段代码的问题在于文件路径中的反斜杠 "\" 需要转义,或者使用原始字符串。可以修改为以下两种方式之一:
1. 双反斜杠转义:
```
df.to_excel("E:\\python-file\\lab3\\Scores.xlsx", index=False)
```
2. 使用原始字符串:
```
df.to_excel(r"E:\python-file\lab3\Scores.xlsx", index=False)
```
另外,还需要确保保存文件的文件夹已经存在,否则会报错。可以先手动创建文件夹,或者使用代码创建文件夹。
阅读全文
相关推荐
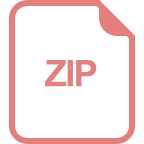
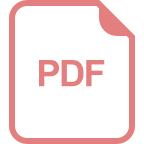









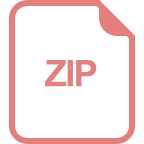
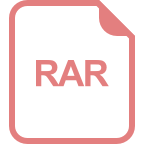




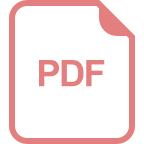