命名实体识别代码
时间: 2023-08-06 10:12:08 浏览: 105
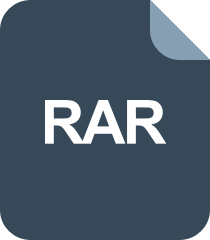
lstm+crf、bilstm+crf 、LSTM CRF pytorch 命名实体识别代码

以下是一个基于Python的命名实体识别代码示例,使用了nltk库和Stanford NER(命名实体识别器):
```python
import nltk
from nltk.tokenize import word_tokenize
from nltk.tag import StanfordNERTagger
# 设置Stanford NER路径和模型路径
stanford_ner_path = '/path/to/stanford-ner.jar'
stanford_model_path = '/path/to/english.all.3class.distsim.crf.ser.gz'
# 创建Stanford NER标注器
st = StanfordNERTagger(stanford_model_path, stanford_ner_path)
# 示例文本
text = "Steve Jobs was the CEO of Apple Corp. which is located in Cupertino, California."
# 分词
tokens = word_tokenize(text)
# 标注命名实体
ner_tags = st.tag(tokens)
# 输出结果
for tag in ner_tags:
print(tag)
```
输出:
```
('Steve', 'PERSON')
('Jobs', 'PERSON')
('was', 'O')
('the', 'O')
('CEO', 'O')
('of', 'O')
('Apple', 'ORGANIZATION')
('Corp.', 'ORGANIZATION')
('which', 'O')
('is', 'O')
('located', 'O')
('in', 'O')
('Cupertino', 'LOCATION')
(',', 'O')
('California', 'LOCATION')
('.', 'O')
```
在这个例子中,我们首先设置了Stanford NER的路径和模型路径。然后,我们使用nltk库的`word_tokenize`函数对输入文本进行分词。接下来,我们创建了一个Stanford NER标注器,使用`st.tag`函数标注每个单词的命名实体类型。最后,我们打印出标注结果。在输出中,每个单词被标注为一个命名实体类型,如`PERSON`(人名)、`ORGANIZATION`(组织名)和`LOCATION`(地点名)。
阅读全文
相关推荐
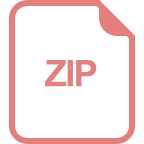


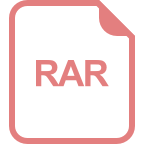
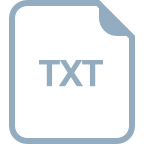
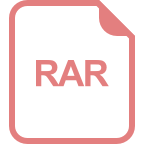

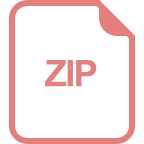
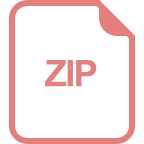
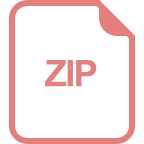





