data = pd.read_excel(inputfile) "Engine should not be specified when passing " "an ExcelFile - ExcelFile already has the engine set"
时间: 2024-03-17 20:42:42 浏览: 28
这个问题可能是因为你传递了 `engine` 参数,而同时又使用了 `ExcelFile` 方法读取 Excel 文件。`ExcelFile` 方法已经包含了一个默认的 `engine` 参数,因此你不需要再传递 `engine` 参数。如果你想指定一个不同的 `engine` 参数,可以使用 `pd.read_excel` 方法来读取 Excel 文件,而不是使用 `ExcelFile` 方法。你可以尝试修改代码,如下所示:
```
import pandas as pd
# 创建 ExcelFile 对象
xls = pd.ExcelFile(inputfile)
# 读取 Excel 文件中的数据
data = pd.read_excel(xls, sheet_name=sheet_name)
```
这样就不需要指定 `engine` 参数了。同时,你可以在 `read_excel` 方法中使用 `sheet_name` 参数来指定要读取的工作表名称。
相关问题
df_1=pd.read_csv('REITs_2013-2019.csv',header=10,index_col=0) df_1[1].drop()
There is a syntax error in the given code. The correct code should be:
```
df_1 = pd.read_csv('REITs_2013-2019.csv', header=10, index_col=0)
df_1 = df_1.drop(1, axis=0)
```
This code reads a CSV file named "REITs_2013-2019.csv" into a pandas DataFrame object called "df_1". The "header=10" parameter specifies that the header row is located at row 10 in the CSV file. The "index_col=0" parameter specifies that the first column in the CSV file should be used as the index (row labels) for the DataFrame.
The second line of code drops the row with index 1 from the DataFrame. The "axis=0" parameter specifies that the row should be dropped (as opposed to dropping a column, which would be specified with "axis=1"). The resulting DataFrame is saved back to the "df_1" variable.
解释import pandas as pd df = pd.read_csv('S12_wearther_central_park.csv') df['DATE'] = pd.to_datetime(df['DATE']) df.set_index('DATE', inplace=True) x = input() year_df = df.loc[str(x), ['PRCP', 'TMIN', 'TMAX']] rainy_days = year_df[year_df['PRCP'] > 1.3] print(rainy_days)
Certainly! Let me explain what each line of the code does:
```python
import pandas as pd
```
This line imports the Pandas library and assigns it the alias `pd`, which is commonly used in Python code.
```python
df = pd.read_csv('S12_wearther_central_park.csv')
```
This line reads the CSV file 'S12_wearther_central_park.csv' into a Pandas DataFrame called `df`. The data in the CSV file is assumed to be comma-separated.
```python
df['DATE'] = pd.to_datetime(df['DATE'])
```
This line converts the 'DATE' column of the DataFrame to a Pandas datetime object. This allows us to perform various operations on the date, such as filtering by year or month.
```python
df.set_index('DATE', inplace=True)
```
This line sets the 'DATE' column as the index of the DataFrame. This is useful for quickly accessing data based on the date.
```python
x = input()
```
This line prompts the user for input and assigns it to the variable `x`. This input is assumed to be a year in the format of a string, e.g. '2010'.
```python
year_df = df.loc[str(x), ['PRCP', 'TMIN', 'TMAX']]
```
This line creates a new DataFrame called `year_df` that contains the precipitation, minimum temperature, and maximum temperature data for the year specified by the user input. The `.loc` method is used to slice the DataFrame by the year, and the square brackets are used to select the columns of interest.
```python
rainy_days = year_df[year_df['PRCP'] > 1.3]
```
This line creates a new DataFrame called `rainy_days` that contains only the rows of `year_df` where the precipitation value is greater than 1.3 inches. This is done by using boolean indexing and comparing the 'PRCP' column to the value 1.3.
```python
print(rainy_days)
```
This line prints the `rainy_days` DataFrame to the console. This DataFrame contains the date, precipitation, minimum temperature, and maximum temperature for the days where precipitation was greater than 1.3 inches.
相关推荐
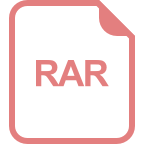
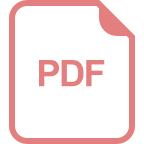
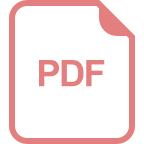













