梯度 图像评价 C++ opencv
时间: 2024-07-06 11:00:33 浏览: 153
梯度图像评价在OpenCV(开源计算机视觉库)中主要用于分析和评估图像中的边缘、纹理和整体结构信息。C++中使用OpenCV进行梯度图像操作通常涉及到以下几个步骤:
1. **计算梯度**:OpenCV的`cv::Mat`类提供了` Sobel()`或`Scharr()`等函数,可以计算图像的一阶或二阶梯度,得到x和y方向的梯度分量。例如,`cv::Sobel(img, gradX, CV_32F, 1, 0)`会计算输入图像`img`的水平梯度。
2. **应用高斯模糊**:为了减少噪声影响,有时会在计算梯度之前对图像应用高斯滤波器,`cv::GaussianBlur()`函数可以做到这一点。
3. **计算模长和方向**:从x和y方向的梯度中,可以计算出梯度的模(强度)和方向(通常用极坐标表示),例如`sqrt(gradX * gradX + gradY * gradY)`。
4. **评估边缘质量**:可以使用像边缘连接性、角点检测(如Harris角点检测)等方法来评估梯度图像的质量。OpenCV也提供了专门的函数,如`cornerHarris()`用于角点检测。
5. **可视化结果**:使用OpenCV的`imshow()`函数显示原始图像、梯度图像、或根据评价结果调整后的图像。
相关问题--
1. 在OpenCV中如何使用高斯模糊预处理图像以提高梯度计算的精度?
2. 除了Sobel和Scharr,OpenCV还提供哪些常用的梯度计算函数?
3. 如何利用OpenCV的角点检测函数评估梯度图像中的特征点?
相关问题
c++ opencv图像处理
C++ OpenCV是一种常用的图像处理库,可以用来进行图像的加载、修改和保存等操作。通过使用OpenCV的函数和方法,可以实现对图像的掩膜操作、对比度调整、均衡化以及形态学操作等。
例如,可以使用imread函数加载图像,如下所示:
```cpp
Mat src = imread("C:\\Users\\td\\Desktop\\he.jpeg");
```
然后,可以使用cvtColor函数将图像转换为指定的色彩空间,例如将BGR色彩空间转换为BGR555色彩空间:
```cpp
Mat output;
cvtColor(src, output,CV_BGR2BGR555);
```
接下来,可以使用imwrite函数保存修改后的图像:
```cpp
imwrite("D:/zhubajie.png", output);
```
除了图像加载和保存,还可以进行其他图像处理操作。例如,可以使用equalizeHist函数对图像进行均衡化处理:
```cpp
cvtColor(src, src, CV_BGR2GRAY);
Mat dst;
equalizeHist(src, dst);
```
还可以使用morphologyEx函数进行形态学操作,如开操作、闭操作、梯度操作等。例如,可以使用getStructuringElement函数创建结构元素,并通过morphologyEx函数对图像进行黑帽操作:
```cpp
Mat kernel = getStructuringElement(MORPH_RECT, Size(11, 11), Point(-1, -1));
morphologyEx(src, dst, CV_MOP_BLACKHAT, kernel);
```
以上是C++ OpenCV图像处理的一些基本操作,根据实际需求可以使用不同的函数和方法来完成不同的图像处理任务。<span class="em">1</span><span class="em">2</span><span class="em">3</span><span class="em">4</span>
c++基于梯度算子(或Robert算子、或Prewitt算子锐化、或Sobel算子锐化)生成梯度图像,基于梯度图像利用边缘跟踪算法跟踪图像里的物体边缘,生成边缘图像。不使用opencv和matlabe
以下是一个 C++ 程序,使用 Sobel 算子进行图像锐化并生成边缘图像,不使用 OpenCV 和 MATLAB:
```c++
#include <iostream>
#include <fstream>
#include <vector>
#include <cmath>
struct Image {
int width;
int height;
std::vector<unsigned char> data;
};
// 从文件中读取 PGM 格式图像
void readPGM(const std::string &filename, Image &image) {
std::ifstream file(filename, std::ios::binary);
if (!file.is_open()) {
std::cerr << "Error: Failed to open file " << filename << std::endl;
exit(1);
}
std::string magicNumber;
file >> magicNumber;
if (magicNumber != "P5") {
std::cerr << "Error: Not a PGM file" << std::endl;
exit(1);
}
file >> image.width;
file >> image.height;
int maxVal;
file >> maxVal;
if (maxVal > 255) {
std::cerr << "Error: Only 8-bit PGM files are supported" << std::endl;
exit(1);
}
file.get(); // 读取换行符
image.data.resize(image.width * image.height);
file.read(reinterpret_cast<char *>(image.data.data()), image.width * image.height);
}
// 保存 PGM 格式图像到文件
void writePGM(const std::string &filename, const Image &image) {
std::ofstream file(filename, std::ios::binary);
if (!file.is_open()) {
std::cerr << "Error: Failed to open file " << filename << std::endl;
exit(1);
}
file << "P5" << std::endl;
file << image.width << " " << image.height << std::endl;
file << "255" << std::endl;
file.write(reinterpret_cast<const char *>(image.data.data()), image.width * image.height);
}
// Sobel 算子计算梯度
void sobel(const Image &input, Image &output) {
int width = input.width;
int height = input.height;
std::vector<int> gx{-1, 0, 1, -2, 0, 2, -1, 0, 1};
std::vector<int> gy{-1, -2, -1, 0, 0, 0, 1, 2, 1};
output.width = width;
output.height = height;
output.data.resize(width * height);
for (int y = 1; y < height - 1; y++) {
for (int x = 1; x < width - 1; x++) {
int sumX = 0;
int sumY = 0;
for (int j = -1; j <= 1; j++) {
for (int i = -1; i <= 1; i++) {
int pixel = static_cast<int>(input.data[(y + j) * width + (x + i)]);
sumX += gx[(j + 1) * 3 + (i + 1)] * pixel;
sumY += gy[(j + 1) * 3 + (i + 1)] * pixel;
}
}
int magnitude = static_cast<int>(std::sqrt(sumX * sumX + sumY * sumY));
output.data[y * width + x] = static_cast<unsigned char>(magnitude);
}
}
}
// 二值化图像
void threshold(const Image &input, Image &output, unsigned char thresholdValue) {
output.width = input.width;
output.height = input.height;
output.data.resize(input.width * input.height);
for (int i = 0; i < input.width * input.height; i++) {
output.data[i] = (input.data[i] >= thresholdValue) ? 255 : 0;
}
}
// 边缘跟踪算法
void edgeTrace(const Image &input, Image &output) {
int width = input.width;
int height = input.height;
output.width = width;
output.height = height;
output.data.resize(width * height);
std::vector<int> dx{-1, 0, 1, -1, 0, 1, -1, 0, 1};
std::vector<int> dy{-1, -1, -1, 0, 0, 0, 1, 1, 1};
for (int y = 1; y < height - 1; y++) {
for (int x = 1; x < width - 1; x++) {
if (input.data[y * width + x] == 0) {
continue;
}
bool isEdge = false;
for (int i = 0; i < 8; i++) {
int neighborX = x + dx[i];
int neighborY = y + dy[i];
if (input.data[neighborY * width + neighborX] == 255) {
isEdge = true;
break;
}
}
output.data[y * width + x] = isEdge ? 255 : 0;
}
}
}
int main(int argc, char *argv[]) {
if (argc != 3) {
std::cerr << "Usage: " << argv[0] << " input_image_path output_edge_image_path" << std::endl;
return 1;
}
// 读取输入图像
std::string inputImagePath = argv[1];
Image inputImage;
readPGM(inputImagePath, inputImage);
// 计算梯度
Image gradientImage;
sobel(inputImage, gradientImage);
// 二值化图像
Image thresholdImage;
threshold(gradientImage, thresholdImage, 128);
// 边缘跟踪
Image edgeImage;
edgeTrace(thresholdImage, edgeImage);
// 保存边缘图像
std::string outputImagePath = argv[2];
writePGM(outputImagePath, edgeImage);
return 0;
}
```
程序实现了 Sobel 算子的计算、图像的二值化和边缘跟踪算法。程序读取 PGM 格式图像,并将结果保存为 PGM 格式的边缘图像。
阅读全文
相关推荐
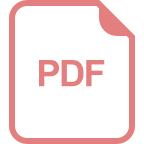
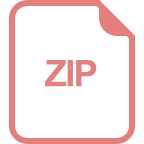
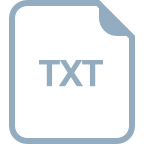
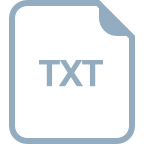
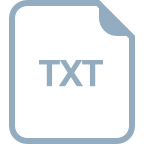
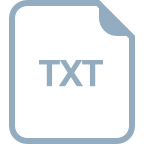
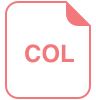








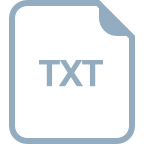
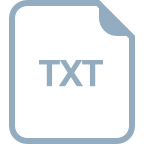