怎么解决from sklearn.metrics import accuracy_score, classification_report, confusion_matrix x_pred=lr.predict(x_train) score1=accuracy_score(y_train,x_pred) y_pred=lr.predict(x_test) score2=accuracy_score(y_test,y_pred) print(score1 , score2)问题 Classification metrics can't handle a mix of binary and continuous targets
时间: 2023-08-18 20:05:30 浏览: 52
根据错误提示,你的目标变量(y_train 和 y_test)是一个混合了二进制和连续值的组合。分类度量标准不能直接应用于这样的情况。
解决这个问题的方法之一是将连续变量转换为二进制变量,以便可以使用分类度量标准。你可以将连续变量转换为二进制变量的方法包括使用阈值进行二元化或者将其转换为分类变量。
例如,假设你的连续变量表示某个数值大于阈值时为正类,小于等于阈值时为负类,你可以使用 `numpy` 库来实现二元化:
```python
import numpy as np
threshold = 0.5 # 设置阈值
y_train_binary = np.where(y_train > threshold, 1, 0) # 将连续变量转换为二进制变量
y_test_binary = np.where(y_test > threshold, 1, 0) # 将连续变量转换为二进制变量
x_pred = lr.predict(x_train)
score1 = accuracy_score(y_train_binary, x_pred)
y_pred = lr.predict(x_test)
score2 = accuracy_score(y_test_binary, y_pred)
print(score1, score2)
```
在上面的代码中,我们使用 `numpy` 的 `where` 函数根据阈值将连续变量转换为二进制变量,并将其用于计算准确率。
请注意,具体的转换方法取决于你的数据和问题的特点,所以你可能需要根据实际情况进行调整。
相关问题
from sklearn.metrics import accuracy_score, classification_report, confusion_matrix score1=accuracy_score(y_train,x_blend) y_pred=meta_model.predict(x_test) score2=accuracy_score(y_test,y_pred) print(score1,score2)报错
这段代码报错的原因可能是由于 `y_train` 和 `x_blend` 的维度不匹配导致无法计算准确率(accuracy)。请确保 `y_train` 和 `x_blend` 都具有相同的样本数量。
另外,如果 `x_blend` 是一个模型的预测结果,它应该是一个一维数组(或列表),而不是多维数组。可以尝试使用 `reshape()` 函数将其转换为一维数组。
以下是一个修正后的示例代码:
```python
from sklearn.metrics import accuracy_score
# 假设 x_blend 是一个二维数组,需要转换为一维数组
x_blend = x_blend.reshape(-1)
score1 = accuracy_score(y_train, x_blend)
y_pred = meta_model.predict(x_test)
score2 = accuracy_score(y_test, y_pred)
print(score1, score2)
```
如果问题仍然存在,请提供完整的错误消息以便我更好地帮助您解决问题。
from sklearn.metrics import accuracy_score, classification_report, confusion_matrix x_pred=meta_model.predict(x_blend) score1=accuracy_score(y_train,x_pred) y_pred=meta_model.predict(x_test) score2=accuracy_score(y_test,y_pred) print(score1 , score2) 出现不连续错误如何代码解决
如果您在使用 `accuracy_score` 函数时遇到 "Found input variables with inconsistent numbers of samples" 的错误,这意味着您的 `x_blend` 和 `y_train` 或者 `x_test` 和 `y_test` 的样本数量不匹配。
要解决这个问题,请确保 `x_blend` 和 `y_train` 以及 `x_test` 和 `y_test` 具有相同的样本数量。您可以使用 `shape` 属性来检查它们的维度。
以下是一个示例代码,用于检查样本数量:
```python
print(x_blend.shape, y_train.shape)
print(x_test.shape, y_test.shape)
```
确保输出的结果中第一个维度(即样本数量)相同。如果不同,您需要重新检查数据处理的步骤,确保正确匹配样本数量。
另外,请注意,在使用 `accuracy_score` 函数时,第一个参数应该是真实标签,而第二个参数应该是预测标签。所以在计算 `score1` 时,应该使用 `y_train` 和 `x_pred`,而在计算 `score2` 时,应该使用 `y_test` 和 `y_pred`,如下所示:
```python
score1 = accuracy_score(y_train, x_pred)
score2 = accuracy_score(y_test, y_pred)
```
确保正确地传递了真实标签和预测标签。
如果问题仍然存在,请提供更多的代码和错误信息,以便我更好地帮助您解决问题。
相关推荐
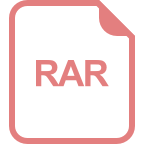











