jupyter最小二乘法
时间: 2023-11-23 07:56:46 浏览: 29
最小二乘法是一种常用的回归分析方法,可以用于求解多元线性回归方程。在jupyter中,可以使用numpy和matplotlib库来实现最小二乘法的计算和可视化。以下是一个简单的例子:
```python
import numpy as np
import matplotlib.pyplot as plt
# 定义数据
x = np.array([1, 2, 3, 4, 5])
y = np.array([2, 3, 5, 6, 8])
# 计算系数
a, b = np.polyfit(x, y, 1)
# 计算预测值
y_hat = a * x + b
# 计算R^2
r_squared = 1 - np.sum((y - y_hat) ** 2) / np.sum((y - np.mean(y)) ** 2)
# 绘制图像
plt.scatter(x, y)
plt.plot(x, y_hat, color='r')
plt.show()
# 输出结果
print("线性回归方程为:y=", a, "x+", b)
print("R^2:", r_squared)
```
在这个例子中,我们使用numpy的`polyfit`函数来计算系数,使用matplotlib的`scatter`和`plot`函数来绘制图像。最后,我们计算R^2并输出结果。
相关问题
jupyter和jupyterlab
JupyterLab 和 Jupyter Notebook 都是用于交互式计算和数据科学的工具,它们都是由 Jupyter 项目开发的。
Jupyter Notebook 是 Jupyter 项目的第一个工具,它提供了一个交互式的编程环境,可以在其中编写和运行代码、展示数据和文本等。Jupyter Notebook 以.ipynb 文件格式保存,每个文件都包含了一些代码块和 Markdown 文本块。
JupyterLab 是 Jupyter 项目的下一代工具,提供了比 Jupyter Notebook 更为强大的功能,如多个文档的编辑、分栏浏览、扩展性等等。JupyterLab 的用户界面更加现代化,可以与其他常见的开发工具和技术集成。
总的来说,JupyterLab 是 Jupyter Notebook 的升级版,提供了更多的功能和更好的用户体验。如果你只是简单地使用 Jupyter Notebook 进行编程和数据科学工作,那么它已经足够好用了。但如果你需要更高级的功能,如多文档编辑和扩展性等,那么 JupyterLab 就是更好的选择。
jupyter 和jupyter notebook
Jupyter和Jupyter Notebook是用于交互式计算和数据科学的工具。Jupyter Notebook是Jupyter项目的早期版本,而JupyterLab是在Jupyter Notebook的基础上进行改进和扩展的工具。
Jupyter Notebook使用基于浏览器的界面,逐个单元格执行代码。它提供了一种以笔记本形式创建、运行和共享代码、文本和可视化结果的方式。
JupyterLab是Jupyter Notebook的升级版本,提供了更现代化和灵活的用户界面,具有更好的可扩展性和集成性。与Jupyter Notebook不同,JupyterLab采用了标签页式的界面布局,允许用户在一个窗口中同时打开多个笔记本、编辑器和终端等组件。它引入了一些新功能和改进,如文件浏览器、集成的代码编辑器和定制化设置。
相关推荐
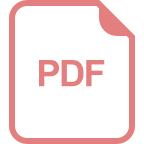












