# Matrix Indexing ----------------------------- A <- matrix(1:6,2,3) A[1,3] A[2,] A[,2] A[1:2,2] A[2,1:2] A[,c(1,3)] A[,-1]
时间: 2023-09-10 11:09:00 浏览: 37
这段代码介绍了 R 语言中的矩阵索引。
在 R 语言中,可以使用方括号 [] 来对矩阵进行索引操作。例如,在代码中,定义了一个 2 行 3 列的矩阵 A。
使用 A[1,3] 可以获取矩阵 A 的第 1 行第 3 列的元素,返回结果为 3。
使用 A[2,] 可以获取矩阵 A 的第 2 行的所有元素,返回结果为向量 c(3, 6)。
使用 A[,2] 可以获取矩阵 A 的第 2 列的所有元素,返回结果为向量 c(2, 5)。
使用 A[1:2,2] 可以获取矩阵 A 的第 1 行和第 2 行的第 2 列元素,返回结果为向量 c(2, 5)。
使用 A[2,1:2] 可以获取矩阵 A 的第 2 行的第 1 列和第 2 列元素,返回结果为向量 c(4, 5)。
使用 A[,c(1,3)] 可以获取矩阵 A 的第 1 列和第 3 列的所有元素,返回结果为一个 2 行 2 列的矩阵。
使用 A[,-1] 可以获取矩阵 A 的除了第 1 列以外的所有元素,返回结果为一个 2 行 2 列的矩阵。
相关问题
add padding of 0 for interpolation window 4x4 and translation [-1,1]
To add padding of 0 for an interpolation window of 4x4 and translation [-1,1], you can use the following code snippet in MATLAB:
```
% Define the interpolation window size and translation
win_size = 4;
translation = [-1, 1];
% Create a padding matrix with zeros
padding = zeros(win_size);
% Compute the indices of the padded region
x_min = win_size/2 - translation(1);
x_max = x_min + win_size - 1;
y_min = win_size/2 - translation(2);
y_max = y_min + win_size - 1;
% Copy the original region to the padded region
padding(x_min:x_max, y_min:y_max) = original_region;
```
In this code snippet, we first define the size of the interpolation window and the translation. We then create a padding matrix with zeros using the "zeros" function in MATLAB. We then compute the indices of the padded region based on the translation and the interpolation window size. Finally, we copy the original region to the padded region by indexing into the "padding" matrix and assigning the values from the "original_region" matrix.
Python库中还有哪些可以实现TF-IDF、TextRank和LSA三种算法的程序包,并通过实例实现关键词提取。
关于TF-IDF、TextRank和LSA三种算法实现关键词提取的Python程序包,常用的包有:
1. sklearn,它提供了TfidfVectorizer和CountVectorizer等工具,可以实现TF-IDF算法。同时,它也提供了LSA和LDA等主题模型算法。
2. nltk,它是Python自然语言处理的一个常用工具库,其中包含了TextRank算法的实现。
3. gensim,它是一个用于处理文本的Python工具库,提供了LSI(Latent Semantic Indexing,潜在语义分析)和LDA等算法实现。
4. jieba,它是一个中文分词工具库,支持TF-IDF算法。
这些工具库都提供了详细的文档和示例,可以很方便地实现关键词提取。例如,使用sklearn的TfidfVectorizer实现TF-IDF算法的关键词提取代码如下:
```
from sklearn.feature_extraction.text import TfidfVectorizer
texts = ['This is a text', 'Another text', 'Yet another text']
vectorizer = TfidfVectorizer()
tfidf_matrix = vectorizer.fit_transform(texts)
feature_names = vectorizer.get_feature_names()
doc = 0
feature_index = tfidf_matrix[doc,:].nonzero()[1]
tfidf_scores = zip(feature_index, [tfidf_matrix[doc,x] for x in feature_index])
top_keywords = sorted(tfidf_scores, key=lambda x: x[1], reverse=True)[:5]
print(top_keywords)
```
这段代码中,首先使用TfidfVectorizer将文本矩阵转换为TF-IDF矩阵,然后通过get_feature_names方法获取特征名列表,使用nonzero方法获取第0个文本的非零元素下标,通过zip将特征下标和对应的TF-IDF分数打包为元组。最后,使用sorted方法将元组按分数从大到小排序,并选择前5个元组,输出作为关键词。
类似地,使用gensim库的LSI算法实现关键词提取的代码如下:
```
from gensim import corpora
from gensim.models import LsiModel
texts = [['This', 'is', 'a', 'text'], ['Another', 'text'], ['Yet', 'another', 'text']]
dictionary = corpora.Dictionary(texts)
corpus = [dictionary.doc2bow(text) for text in texts]
lsi_model = LsiModel(corpus, num_topics=2)
lsi_matrix = lsi_model[corpus]
doc = 0
top_keywords = sorted(lsi_matrix[doc], key=lambda x: -x[1])[:5]
print(top_keywords)
```
这段代码中,首先使用corpora.Dictionary将文本列表转换为词典,再使用doc2bow方法将每个文本转换为词袋向量表示。然后,使用LsiModel训练得到一个2维的LSI模型,对文本矩阵进行转换得到LSI矩阵。最后,使用sorted方法将LSI矩阵中第0个文本的元素按LSI分数从大到小排序,并选择前5个元素,输出作为关键词。
使用这些工具库,可以很方便地实现关键词提取并进行文本分析。
相关推荐
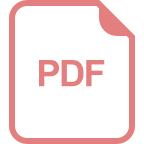












