# 将 OpenCV 格式的图片转换为 PIL.Image 格式 img_pil = Image.fromarray(cv2.cvtColor(copyfile, cv2.COLOR_BGR2RGB)) # 在指定路径中保存图片 if not os.path.exists(os.path.join(rectangle_dir,image_name)): os.makedirs(os.path.join(rectangle_dir,image_name)) image_path = image_name.split('/')[:2] image_real_name=image_name.split('/')[-1] img_pil.save(os.path.join(rectangle_dir,image_name),format='JPEG') IsADirectoryError: [Errno 21] Is a directory: '/home/u1/ywj/deepfashion/clothes_change_ywj/rectangleimg/img/Sheer_Pleated-Front_Blouse/img_00000001.jpg'
时间: 2024-03-25 09:39:28 浏览: 121
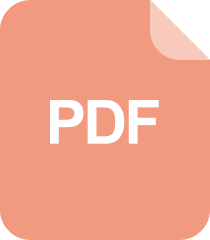
Python 实现OpenCV格式和PIL.Image格式互转
这个错误提示显示在保存图片的时候出现了一个 `IsADirectoryError`,原因是你在调用 `os.makedirs()` 方法创建保存图片的目录时,传入的是 `os.path.join(rectangle_dir,image_name)`,这个路径是包含文件名的完整路径,不应当作为目录路径传入 `os.makedirs()` 方法中。
你可以将路径拆分成目录路径和文件名,然后将目录路径作为参数传入 `os.makedirs()` 方法中,这样就可以创建目录了。然后在保存图片时,只需将目录路径和文件名拼接起来即可。
具体代码如下:
```
# 将 OpenCV 格式的图片转换为 PIL.Image 格式
img_pil = Image.fromarray(cv2.cvtColor(copyfile, cv2.COLOR_BGR2RGB))
# 在指定路径中保存图片
if not os.path.exists(os.path.join(rectangle_dir, image_name)):
# 拆分路径为目录路径和文件名
path_list = image_name.split('/')[:2]
dir_path = os.path.join(rectangle_dir, '/'.join(path_list))
file_name = image_name.split('/')[-1]
# 创建目录
if not os.path.exists(dir_path):
os.makedirs(dir_path)
# 拼接保存图片的路径和文件名
save_path = os.path.join(dir_path, file_name)
# 保存图片
img_pil.save(save_path, format='JPEG')
```
这里使用 `split()` 方法将图片的路径拆分成目录路径和文件名。然后使用 `os.path.join()` 方法将目录路径和 `rectangle_dir` 拼接成完整的目录路径 `dir_path`,并使用 `os.makedirs()` 方法创建目录。最后将目录路径和文件名拼接起来,得到保存图片的完整路径,并调用 `Image.save()` 方法保存图片。
阅读全文
相关推荐
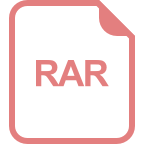















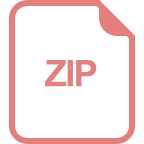