请用pytorch实现一个加入attention机制的RNN
时间: 2023-06-10 13:08:29 浏览: 52
好的,以下是用PyTorch实现一个带有注意力机制的RNN的代码示例:
```
import torch
import torch.nn as nn
class AttentionRNN(nn.Module):
def __init__(self, input_dim, hidden_dim, output_dim):
super(AttentionRNN, self).__init__()
self.hidden_dim = hidden_dim
self.i2h = nn.Linear(input_dim, hidden_dim)
self.h2h = nn.Linear(hidden_dim, hidden_dim)
self.h2o = nn.Linear(hidden_dim, output_dim)
self.attention = nn.Linear(hidden_dim, hidden_dim)
self.v = nn.Linear(hidden_dim, 1, bias=False)
def forward(self, inputs, hidden):
embedded = inputs.unsqueeze(0)
h_t_1 = hidden
lstm_t = self.i2h(embedded) + self.h2h(h_t_1)
lstm_t = torch.tanh(lstm_t)
attn_t = self.attention(lstm_t)
attn_t = torch.tanh(attn_t)
attn_t = self.v(attn_t)
attn_t = torch.softmax(attn_t, dim=0)
attn_lstm_t = (lstm_t * attn_t).sum(dim=0)
o_t = self.h2o(attn_lstm_t)
h_t = attn_lstm_t
return o_t, h_t
def init_hidden(self):
return torch.zeros(1, self.hidden_dim)
```
在这个模型中,我们使用一个带有注意力机制的RNN来处理输入,其中指定了输入维度(`input_dim`), 隐藏层维度(`hidden_dim`)和输出维度(`output_dim`)。
在`forward`函数中,我们首先将当前输入(`inputs`)传递给线性层(`i2h`)来计算LSTM的输入。接下来,我们将上一个时间步的隐藏状态(`hidden`)和当前输入一起传递给另一个线性层(`h2h`)来计算LSTM的隐藏状态。这两个值相加,经过一个tanh激活层后,得到LSTM的输出。
然后,在计算输出之前,我们计算一个“注意力向量”,用于决定哪些部分的输入是最重要的。我们首先使用另一个线性层来将LSTM的输出投影到一个向量空间中。这个向量被传递到一个tanh激活层和一个线性层中,用于计算softmax分数。这些分数用于计算输入的加权和,得到一个最终的向量,我们将其乘以另一个线性层来计算模型的输出。
最后,在初始化隐藏状态时,我们只需返回一个包含所有元素为零的张量,其形状与隐藏层维度(`hidden_dim`)相同。
希望这个代码示例对你有帮助!
相关推荐
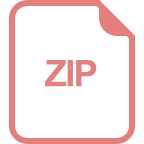














