with open(output_file, "w", encoding="utf-8") as f: for word in text_new =: f.write(word + " ") # 将分词结果写入文件,以空格分隔
时间: 2024-01-03 14:04:03 浏览: 17
在你的代码中,似乎有一个语法错误,`text_new =`后面没有给出一个具体的值。如果你想将`text_new`中的内容写入文件,你可以使用以下代码:
```python
with open(output_file, "w", encoding="utf-8") as f:
f.write(" ".join(text_new)) # 将分词结果写入文件,以空格分隔
```
在这个修改后的代码中,我们使用`" ".join(text_new)`将`text_new`列表中的分词结果用空格连接起来,并将连接后的结果写入文件。
请记得在运行代码之前,确保`text_new`变量中包含了正确的分词结果。此外,还要注意打开文件时使用的编码方式要与写入时指定的编码方式保持一致,这里使用的是`"utf-8"`编码。
相关问题
with open(output_file, "w", encoding="utf-8") as f: f.write("".join(text_new)) # 将分词结果写入文件,以空格分隔
这段代码是将分词结果写入文件的操作。假设你有一个名为output_file的文件路径,并且已经将分词结果保存在text_new列表中。
代码中使用了open函数打开output_file文件,并指定以写入模式("w")打开,同时设置编码为utf-8。接下来使用文件对象f的write方法,将分词结果写入文件中。通过"".join(text_new)将text_new列表中的分词结果按照空字符串连接起来,以空格进行分隔。最后关闭文件对象。
这样,分词结果就会被写入到指定的文件中。
encoding=utf-8 import nltk import json from nltk.corpus import stopwords import re eg_stop_words = set(stopwords.words('english')) sp_stop_words = set(stopwords.words('spanish')) all_stop_words = eg_stop_words.union(sp_stop_words) input_file_name = r'建模.txt' output_file_name = r'train.txt' out_file = open(output_file_name, encoding='utf-8', mode='w') 打开输出文件 with open(output_file_name, encoding='utf-8', mode='w') as output_file: # 打开输入文件,对每一行进行处理 with open(input_file_name, encoding='utf-8') as f: for idx, line in enumerate(f): print("正在处理第{}行数据".format(idx)) if idx == 0: # 第一行是列名, 不要 print(line) continue line = line.strip() sps = line.split("\t") # 将行按制表符分隔为列表 report_no = sps[0] target = sps[2] smses = sps[-1] smses = smses.strip(""") # 去掉短信两端的引号 smses = smses.replace("""", """) # 把两个双引号转换成单引号 root = json.loads(smses) # 解析 json 格式的短信 msg = "" for item in root: # 遍历短信中的每一条信息 body = item["body"] # 获取信息的正文 msg += body + "\n" # 把正文追加到总的信息传递过来的msg中 text = re.sub(r'[^\w\s]', '', msg) # 使用正则表达式去掉标点符号 text = re.sub(r'http\S+', '', text) # 去掉链接 text = re.sub(r'\d+', '', text)#去除数字 text = text.lower() words = text.split() filtered_words = [word for word in words if word not in all_stop_words] text = ' '.join(filtered_words) print(report_no + '\t' + target) msg = target + '\u0001' + text + '\n' out_file.write(msg) out_file.close()帮我改成用 pandas 处理
当使用 pandas 进行处理时,你可以使用 `pandas.read_csv()` 函数读取文件,并使用 DataFrame 进行数据处理。以下是使用 pandas 进行处理的示例代码:
```python
import pandas as pd
import nltk
from nltk.corpus import stopwords
import re
eg_stop_words = set(stopwords.words('english'))
sp_stop_words = set(stopwords.words('spanish'))
all_stop_words = eg_stop_words.union(sp_stop_words)
input_file_name = '建模.txt'
output_file_name = 'train.txt'
df = pd.read_csv(input_file_name, encoding='utf-8', sep='\t')
# 处理每一行数据
for idx, row in df.iterrows():
print("正在处理第{}行数据".format(idx))
if idx == 0:
# 第一行是列名,不需要处理
continue
smses = row['短信']
smses = smses.strip("'")
smses = smses.replace('""', "'")
root = json.loads(smses)
msg = ""
for item in root:
body = item["body"]
msg += body + "\n"
text = re.sub(r'[^\w\s]', '', msg)
text = re.sub(r'http\S+', '', text)
text = re.sub(r'\d+', '', text)
text = text.lower()
words = text.split()
filtered_words = [word for word in words if word not in all_stop_words]
text = ' '.join(filtered_words)
df.at[idx, '目标'] = row['目标'] + '\u0001' + text
df.to_csv(output_file_name, encoding='utf-8', sep='\t', index=False)
```
这段代码将会读取名为 '建模.txt' 的文件,并根据每一行的数据进行处理,最后将处理后的结果保存到 'train.txt' 文件中。注意在使用该代码前,你需要先安装 pandas 和 nltk 库。
相关推荐
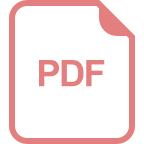
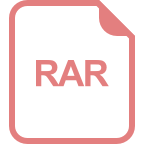
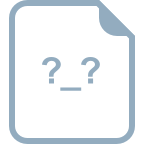













