不调用外部库,在数据集上完成线性回归分类模型的编写与训练
时间: 2024-10-19 19:06:19 浏览: 15
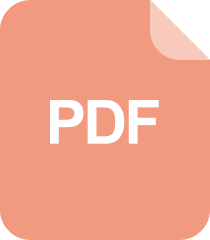
TensorFlow实现iris数据集线性回归
在Python中,如果你想要不依赖外部库(比如Scikit-Learn等机器学习库),你可以手动编写一个简单的线性回归模型来进行分类任务。线性回归通常用于预测连续值,但它通过一些技巧可以转化为分类模型,例如Sigmoid函数将其转换到0到1之间,然后设定阈值将结果二分类。
以下是一个简化的步骤:
1. **创建基础结构**:
- 定义模型类,包含一个权重向量和一个偏置项作为参数。
```python
class LinearRegressionForClassification:
def __init__(self):
self.weights = None
self.bias = None
```
2. **计算预测值**:
- 设定一个sigmoid函数来实现线性回归的“分类”效果。
```python
def predict(self, x):
z = np.dot(x, self.weights) + self.bias
return 1 / (1 + np.exp(-z))
```
3. **初始化参数**:
- 可以随机初始化权重和偏置,也可以使用梯度下降等优化算法逐步调整。
```python
def init_params(self, data, labels):
# 初始化权重和偏置,这里假设数据已经归一化
n_features = data.shape[1]
self.weights = np.random.randn(n_features)
self.bias = 0
```
4. **训练模型**:
- 使用梯度下降或其他优化算法更新权重和偏置,这里仅做演示,未包括迭代和优化过程。
```python
def train(self, data, labels, learning_rate=0.01, epochs=1000):
for epoch in range(epochs):
# 简单的批量梯度下降
predictions = self.predict(data)
d_weights = (data.T @ (predictions - labels)) * (predictions * (1 - predictions))
d_bias = sum(predictions - labels)
self.weights -= learning_rate * d_weights
self.bias -= learning_rate * d_bias
```
5. **评估模型**:
- 需要自行编写交叉验证、准确率等评估指标。
注意:这个示例非常简化,并不适合大规模的实际应用。在实际项目中,你会需要处理更多细节,如正则化、损失函数、批量大小等,并且可能需要使用更成熟的优化库如NumPy、TensorFlow或PyTorch。
阅读全文
相关推荐
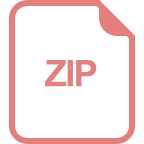
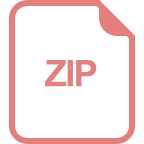


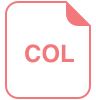
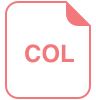
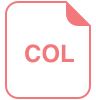
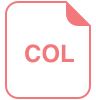
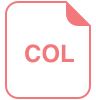
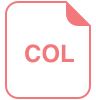
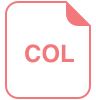
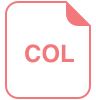
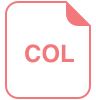
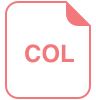
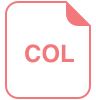
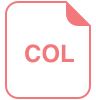
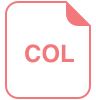
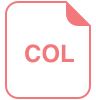