ValueError Traceback (most recent call last) ~\AppData\Local\Temp/ipykernel_8036/2067320651.py in <module> 16 # 对Recency、Frequency、Monetary进行分段打分 17 recency_bins = pd.qcut(df['Recency'], q=5, labels=False) ---> 18 frequency_bins = pd.qcut(df['提交日期_frequency'], q=5, labels=False) 19 monetary_bins = pd.qcut(df['订单金额_monetary'], q=5, labels=False) 20 C:\anaconda\lib\site-packages\pandas\core\reshape\tile.py in qcut(x, q, labels, retbins, precision, duplicates) 362 """ 363 original = x --> 364 x = _preprocess_for_cut(x) 365 x, dtype = _coerce_to_type(x) 366 C:\anaconda\lib\site-packages\pandas\core\reshape\tile.py in _preprocess_for_cut(x) 592 x = np.asarray(x) 593 if x.ndim != 1: --> 594 raise ValueError("Input array must be 1 dimensional") 595 596 return x ValueError: Input array must be 1 dimensional
时间: 2024-04-16 13:26:22 浏览: 145
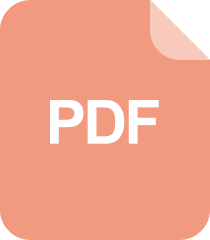
linux 下 pip3 报错“ File “/usr/bin/pip3”, line 9, in from pip import mainImportError: canno”处理

根据错误提示,看起来是因为`pd.qcut()`函数要求输入的数组必须是一维的,而你的`df['提交日期_frequency']`可能是二维的。请确保`df['提交日期_frequency']`是一个一维的Series或DataFrame列。
你可以尝试使用`.squeeze()`方法将其转换为一维的Series,例如:
```python
frequency_bins = pd.qcut(df['提交日期_frequency'].squeeze(), q=5, labels=False)
```
如果`df['提交日期_frequency']`本身就是一个DataFrame列,而不是多列DataFrame,则不需要使用`.squeeze()`方法。
如果问题仍然存在,请提供更多关于你的数据结构和示例数据的信息,以便进一步帮助你解决问题。
阅读全文
相关推荐
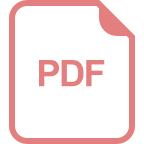
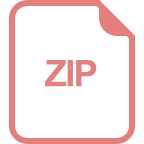
















