AttributeError: 'SVC' object has no attribute '_probA'. Did you mean: 'probA_'?
时间: 2024-02-27 13:50:24 浏览: 59
AttributeError: 'SVC' object has no attribute '_probA' 是一个错误提示,它表示在SVC对象中没有名为'_probA'的属性。它还提供了一个可能的替代选项'probA_'。
这个错误通常发生在使用SVC对象时,尝试访问'_probA'属性,但该属性不存在。可能的原因是:
1. 你可能错误地拼写了属性名。你可以尝试使用'probA_'作为替代选项。
2. 你可能正在使用的是不支持'_probA'属性的版本的SVC对象。你可以检查你所使用的库或框架的文档,确认该属性是否存在。
如果你能提供更多的上下文或代码示例,我可以给出更具体的解答。
相关问题
AttributeError: CoxPHFitter has no attribute 'predict_proba'
这个错误是因为 CoxPHFitter 模型没有 predict_proba 方法。CoxPHFitter 模型是一个用于生存分析的模型,它预测的是事件(如死亡)发生的时间,而不是二分类或多分类问题。因此,它没有预测概率的方法,只有预测事件时间的方法。如果您需要预测概率,可以考虑使用其他模型,如 Logistic 回归或随机森林等。
AttributeError: 'LogitResults' object has no attribute 'predict_proba'
根据提供的引用内容,报错"AttributeError: 'LogitResults' object has no attribute 'predict_proba'"是由于`LogitResults`对象没有`predict_proba`属性导致的。这个错误通常发生在使用旧版本的库或模型时,因为`predict_proba`方法在较新的版本中可能已被删除或更改。
解决这个问题的方法是使用其他可用的方法来获取预测概率。你可以尝试以下方法之一来解决这个问题:
1. 使用`predict`方法代替`predict_proba`方法。`predict`方法通常返回类别的预测结果,而不是概率。你可以根据需要进行调整。
2. 检查你正在使用的库或模型的文档,查看是否有其他方法可以用于获取预测概率。有时候,库或模型可能已经更改了方法的名称或实现方式。
3. 更新你的库或模型到较新的版本,以确保你可以使用最新的方法和功能。这样可以避免由于版本不匹配而导致的错误。
下面是一个示例代码,演示如何使用`predict`方法来获取预测结果:
```python
# 假设你的模型对象是logit_results
predictions = logit_results.predict(X_test) # 使用predict方法获取预测结果
```
相关推荐
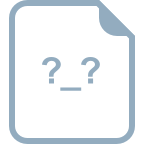
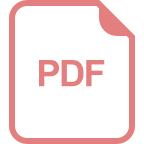
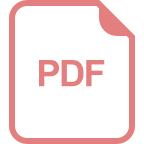












