train loss交叉
时间: 2023-09-24 21:10:24 浏览: 74
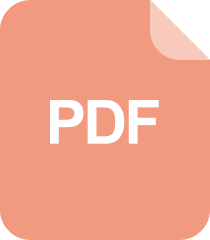
sklearn和keras的数据切分与交叉验证的实例详解
交叉熵损失(Cross-Entropy Loss)是深度学习中常用的一种损失函数,特别适用于多分类任务。交叉熵损失可以用来衡量模型的预测值与真实标签之间的距离,也就是模型预测的概率分布与真实标签的概率分布之间的差异。
在训练神经网络时,我们通常会使用批量梯度下降(Batch Gradient Descent)来更新模型参数。每次迭代更新参数时,我们需要计算模型在当前批量数据上的损失值。对于多分类任务,我们可以使用交叉熵损失作为损失函数,通过最小化交叉熵损失来优化模型参数。
交叉熵损失的公式如下:
$$
\text{Loss} = -\frac{1}{N}\sum_{i=1}^{N}\sum_{j=1}^{C} y_{ij} \log(p_{ij})
$$
其中,$N$ 表示样本总数,$C$ 表示类别数,$y_{ij}$ 表示第 $i$ 个样本的第 $j$ 类标签(如果该样本属于第 $j$ 类,则 $y_{ij}=1$,否则 $y_{ij}=0$),$p_{ij}$ 表示第 $i$ 个样本属于第 $j$ 类的概率。交叉熵损失的含义是,对于每个样本,我们将其真实标签的概率取一个对数,并乘以其对应的预测概率,然后将所有样本的结果累加起来并取相反数。
在训练神经网络时,我们通常会计算一个批量数据上的平均交叉熵损失,然后使用反向传播算法计算梯度并更新模型参数。这样可以有效地提高模型的准确率和泛化性能。
阅读全文
相关推荐
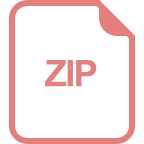
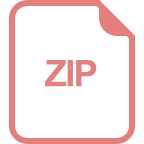
















