emd分解 python
时间: 2023-06-21 12:18:53 浏览: 118
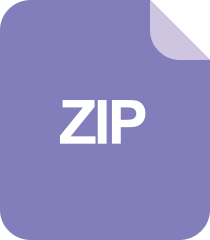
emd分解代码
EMD(Earth Mover's Distance)分解是一种用于图像处理和计算机视觉等领域的算法,用于将两个图像之间的距离分解成一系列基础距离的加权和。在Python中,可以使用PyEMD库来实现EMD分解。
以下是一个简单的示例代码:
```python
from pyemd import emd
import numpy as np
# 定义两个图像的特征向量
hist1 = np.array([0.1, 0.2, 0.3, 0.4])
hist2 = np.array([0.3, 0.3, 0.2, 0.2])
# 定义两个图像之间的基础距离矩阵
D = np.array([[0, 1, 2, 3],
[1, 0, 1, 2],
[2, 1, 0, 1],
[3, 2, 1, 0]])
# 使用EMD分解计算两个图像之间的距离
dist = emd(hist1, hist2, D)
print(dist)
```
在上面的示例代码中,我们首先定义了两个图像的特征向量(hist1和hist2),然后定义了两个图像之间的基础距离矩阵(D)。最后,我们使用EMD函数计算了两个图像之间的距离,并将结果打印出来。
阅读全文
相关推荐
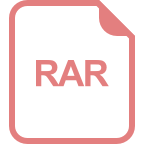



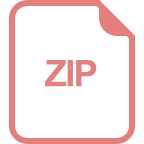


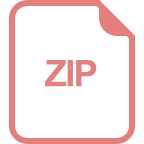






