翻译代码def resize(): img_path = "Normalresult" save_path = "result" img_names = os.listdir(img_path) # 以列表的形式获取文件夹中的所有文件的名字和格式(例如:0.jpg) for img_name in img_names: img = os.path.join(img_path, img_name) # 将文件的绝对路径与每个文件名字进行拼接,以获取该文件 img = cv2.imread(img) # 读取该文件(图片) # 一是通过设置图像缩放比例,即缩放因子,来对图像进行放大或缩小 res1 = cv2.resize(img, None, fx=2, fy=2, interpolation=cv2.INTER_LINEAR) height, width = img.shape[:2] # 二是直接设置图像的大小,不需要缩放因子 res2 = cv2.resize(img, (int(0.8 * width), int(0.8 * height)), interpolation=cv2.INTER_AREA) dir_name1 = ''.join(random.sample(string.ascii_letters + string.digits, 8)) dir_name2 = ''.join(random.sample(string.ascii_letters + string.digits, 8)) cv2.imwrite(os.path.join(save_path, dir_name1 + '.jpg'), res1) cv2.imwrite(os.path.join(save_path, dir_name2 + '.jpg'), res2) cv2.imwrite(os.path.join(save_path, img_name), img)
时间: 2024-04-06 12:29:47 浏览: 18
这段代码的作用是对指定路径下的所有图片进行缩放,并将缩放后的图片保存到指定的保存路径中。具体来说,代码首先使用 os 库中的 listdir 函数获取指定路径下所有图片的文件名,并使用循环遍历每个图片文件名。在循环中,代码使用 os 库中的 join 函数将指定路径和当前图片文件名拼接成完整的路径,并使用 cv2 库中的 imread 函数读取当前图片。
接着,代码使用 cv2 库中的 resize 函数对当前图片进行两种缩放方式的处理。第一种方式是通过设置缩放因子 fx 和 fy 来对图像进行放大或缩小,得到缩放后的图像 res1。第二种方式是直接设置缩放后的图像的大小,得到缩放后的图像 res2。
然后,代码使用 random 和 string 库中的函数生成随机的文件名,并使用 cv2 库中的 imwrite 函数将缩放后的图像 res1 和 res2 以及原始图像 img 分别保存到指定的保存路径中。最后,代码继续循环处理下一个图片。
相关问题
代码import os import numpy as np import nibabel as nib from PIL import Image # 创建保存路径 save_path = 'C:/Users/Administrator/Desktop/2D-LiTS2017' if not os.path.exists(save_path): os.makedirs(save_path) if not os.path.exists(os.path.join(save_path, 'image')): os.makedirs(os.path.join(save_path, 'image')) if not os.path.exists(os.path.join(save_path, 'label')): os.makedirs(os.path.join(save_path, 'label')) # 加载数据集 data_path = 'D:/BaiduNetdiskDownload/LiTS2017' img_path = os.path.join(data_path, 'Training Batch 1') label_path = os.path.join(data_path, 'Training Batch 2') # 转换图像 for file in sorted(os.listdir(img_path)): if file.endswith('.nii'): img_file = os.path.join(img_path, file) img = nib.load(img_file).get_fdata() img = np.transpose(img, (2, 0, 1)) # 转换为z, x, y for i in range(img.shape[0]): img_slice = img[i, :, :] img_slice = (img_slice - np.min(img_slice)) / (np.max(img_slice) - np.min(img_slice)) * 255 # 归一化到0-255 img_slice = img_slice.astype(np.uint8) img_slice = np.stack([img_slice]*3, axis=2) # 转换为三通道图像 img_name = file[:-4] + '' + str(i).zfill(3) + '.png' img_file_save = os.path.join(save_path, 'image', img_name) Image.fromarray(img_slice).save(img_file_save) # 转换标签 for file in sorted(os.listdir(label_path)): if file.endswith('.nii'): label_file = os.path.join(label_path, file) label = nib.load(label_file).get_fdata() label = np.transpose(label, (2, 0, 1)) # 转换为z, x, y for i in range(label.shape[0]): label_slice = label[i, :, :] label_slice[label_slice == 1] = 255 # 肝脏灰度值设为255 label_slice[label_slice == 2] = 128 # 肝脏肿瘤灰度值设为128 label_slice = label_slice.astype(np.uint8) label_name = file[:-4] + '' + str(i).zfill(3) + '.png' label_file_save = os.path.join(save_path, 'label', label_name) Image.fromarray(label_slice).save(label_file_save)出现scaled = scaled.astype(np.promote_types(scaled.dtype, dtype), copy=False) MemoryError错误,怎么修改?给出完整代码
这个错误通常意味着你的计算机没有足够的内存来处理数组。可能需要减少转换图像的尺寸或者使用更高效的算法。以下是一个可能的修改建议:
```python
import os
import numpy as np
import nibabel as nib
from PIL import Image
# 创建保存路径
save_path = 'C:/Users/Administrator/Desktop/2D-LiTS2017'
if not os.path.exists(save_path):
os.makedirs(save_path)
if not os.path.exists(os.path.join(save_path, 'image')):
os.makedirs(os.path.join(save_path, 'image'))
if not os.path.exists(os.path.join(save_path, 'label')):
os.makedirs(os.path.join(save_path, 'label'))
# 加载数据集
data_path = 'D:/BaiduNetdiskDownload/LiTS2017'
img_path = os.path.join(data_path, 'Training Batch 1')
label_path = os.path.join(data_path, 'Training Batch 2')
# 转换图像
for file in sorted(os.listdir(img_path)):
if file.endswith('.nii'):
img_file = os.path.join(img_path, file)
img = nib.load(img_file).get_fdata()
img = np.transpose(img, (2, 0, 1)) # 转换为z, x, y
for i in range(img.shape[0]):
img_slice = img[i, :, :]
img_slice = (img_slice - np.min(img_slice)) / (np.max(img_slice) - np.min(img_slice)) * 255 # 归一化到0-255
img_slice = img_slice.astype(np.uint8)
img_slice = np.stack([img_slice]*3, axis=2) # 转换为三通道图像
#减少图像大小
img_slice = np.array(Image.fromarray(img_slice).resize((512, 512), resample=Image.BICUBIC))
img_name = file[:-4] + '' + str(i).zfill(3) + '.png'
img_file_save = os.path.join(save_path, 'image', img_name)
Image.fromarray(img_slice).save(img_file_save)
# 转换标签
for file in sorted(os.listdir(label_path)):
if file.endswith('.nii'):
label_file = os.path.join(label_path, file)
label = nib.load(label_file).get_fdata()
label = np.transpose(label, (2, 0, 1)) # 转换为z, x, y
for i in range(label.shape[0]):
label_slice = label[i, :, :]
label_slice[label_slice == 1] = 255 # 肝脏灰度值设为255
label_slice[label_slice == 2] = 128 # 肝脏肿瘤灰度值设为128
label_slice = label_slice.astype(np.uint8)
label_name = file[:-4] + '' + str(i).zfill(3) + '.png'
label_file_save = os.path.join(save_path, 'label', label_name)
Image.fromarray(label_slice).save(label_file_save)
```
这里我们使用了 `PIL.Image` 中的 `resize()` 函数来减少图像大小。`resample=Image.BICUBIC` 用于指定插值方法,这里使用了 Bicubic 插值。这个方法可以保留图像的细节,并且可以减少内存使用。你也可以尝试使用其他插值方法。
# 加载数据集 data_dir =r"C:\Users\HP\Desktop\image\save" patients = os.listdir(data_dir) images = [] for patient in patients: patient_dir = os.path.join(data_dir, patient) imgs = os.listdir(patient_dir) for img_name in imgs: img_path = os.path.join(patient_dir, img_name) img = cv2.imread(img_path) img_gray = cv2.cvtColor(img, cv2.COLOR_BGR2GRAY) img_gray = cv2.resize(img_gray, (256, 256)) img_gray = img_gray / 255.0 images.append(img_gray) images = np.array(images) images = np.reshape(images, (-1, 256, 256, 1)) 如何修改这段代码使得这段代码可以读取save文件夹下面所有病人的图片
您可以使用 os.walk() 函数来遍历 save 文件夹下的所有子文件夹和文件,然后根据文件名中的信息来判断是否是病人的图片。以下是修改后的代码:
```
import os
import cv2
import numpy as np
data_dir = r"C:\Users\HP\Desktop\image\save"
images = []
for root, dirs, files in os.walk(data_dir):
for file in files:
if "patient" in file: # 判断文件名中是否包含病人信息
img_path = os.path.join(root, file)
img = cv2.imread(img_path)
img_gray = cv2.cvtColor(img, cv2.COLOR_BGR2GRAY)
img_gray = cv2.resize(img_gray, (256, 256))
img_gray = img_gray / 255.0
images.append(img_gray)
images = np.array(images)
images = np.reshape(images, (-1, 256, 256, 1))
```
这样可以读取 save 文件夹下所有病人的图片,不论这些图片在哪个子文件夹下。
相关推荐
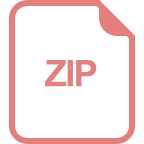
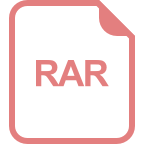













