粗糙集属性约简方法与python实现
时间: 2024-11-09 14:26:40 浏览: 32
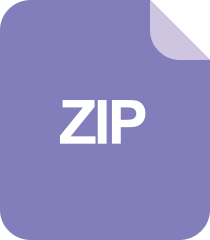
邻域粗糙集属性约简,粗糙集属性约简步骤,Python

粗糙集理论是一种数据挖掘和知识发现的技术,它基于信息系统的不确定性和不完备性来进行决策分析。其中,属性约简是一个关键步骤,目的是从原始属性集中选择出对分类最有影响力的属性集合,简化模型的同时保持预测性能。
粗糙集的属性约简方法主要包括:
1. **基于信息增益的约简**:如ID3算法,通过计算属性的信息熵减少不确定性。
2. **基于置信度的约简**:如Rough Set-Based Attribute Reduction (RSBAR)方法,考虑了属性值的支持度和置信度。
3. **基于粗糙集下界估计的约简**:如LCM (Lift and Compress) 方法,寻找最小的支持度下界。
Python有多种库可以支持粗糙集分析,比如`pyroughsets`、`rscikit`等。例如,使用`pyroughsets`库,你可以编写类似这样的代码:
```python
from pyroughsets import RAS, InfoGain
# 假设你有一个DataFrame df,包含属性集X和目标变量Y
ras = RAS(df, attribute_col='X', class_label='Y')
attribute_reduction = ras.reduce_by_entropy() # 使用信息增益进行约简
# 查看约简后的属性集
reduced_attributes = attribute_reduction['Attributes']
```
阅读全文
相关推荐
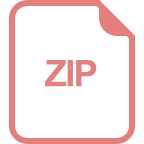
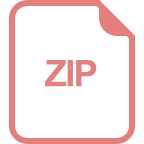














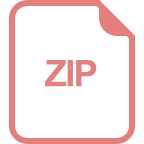