def correct_colours(im1, im2, landmarks1): blur_amount = COLOUR_CORRECT_BLUR_FRAC * numpy.linalg.norm( numpy.mean(landmarks1[LEFT_EYE_POINTS], axis=0) - numpy.mean(landmarks1[RIGHT_EYE_POINTS], axis=0)) blur_amount = int(blur_amount) if blur_amount % 2 == 0: blur_amount += 1 im1_blur = cv2.GaussianBlur(im1, (blur_amount, blur_amount), 0) im2_blur = cv2.GaussianBlur(im2, (blur_amount, blur_amount), 0) # 避免被零除的错误。 im2_blur += (128 * (im2_blur <= 1.0)).astype(im2_blur.dtype) return (im2.astype(numpy.float64) * im1_blur.astype(numpy.float64) / im2_blur.astype(numpy.float64))
时间: 2023-12-27 12:02:35 浏览: 49
这段代码的作用是对两张图像进行颜色校正,使它们具有相似的颜色分布。它的输入参数为两张图像(im1和im2)和第一张图像的关键点(landmarks1)。其中,通过计算左右眼关键点的平均值,确定了高斯模糊的半径,然后对两张图像进行高斯模糊。接着,避免了被零除的错误,最后将im2校正为与im1具有相似颜色分布的图像。
相关问题
import cv2 import numpy as np def CannyThreshold(lowThreshold): detected_edges = cv2.GaussianBlur(gray,(3,3),0) detected_edges = cv2.Canny(detected_edges,lowThreshold,lowThreshold*ratio,apertureSize = kernel_size) dst = cv2.bitwise_and(img,img,mask = detected_edges) # just add some colours to edges from original image. cv2.imshow('canny demo',dst) lowThreshold = 0 max_lowThreshold = 300 #最大阈值 ratio = 3 kernel_size = 3 #img = cv2.imread('AAA.jpg', cv2.IMREAD_GRAYSCALE) #注释此行图片不灰度化 img = cv2.imread("D:\xiao.jpg") #注释此行上行代码不注释图片灰度化 gray = cv2.imread("D:\xiao.jpg", cv2.IMREAD_GRAYSCALE) cv2.namedWindow('canny demo') cv2.createTrackbar('Min threshold','canny demo',lowThreshold, max_lowThreshold, CannyThreshold) CannyThreshold(0) # initialization if cv2.waitKey(0) == 27: cv2.destroyAllWindows()
这段代码是使用OpenCV库进行Canny边缘检测的操作,并且使用了滑动条来调节最小阈值。其中,首先对输入的图像进行了高斯模糊处理,然后使用Canny函数进行边缘检测,最后使用bitwise_and函数将原图像和检测出的边缘进行合并并显示出来。滑动条的回调函数是CannyThreshold,用于实时更新最小阈值并重新进行边缘检测和显示。如果按下ESC键,则关闭窗口。
分析错误import cv2 import numpy as np def CannyThreshold(lowThreshold): detected_edges = cv2.GaussianBlur(gray,(3,3),0) detected_edges = cv2.Canny(detected_edges,lowThreshold,lowThreshold*ratio,apertureSize = kernel_size) dst = cv2.bitwise_and(img,img,mask = detected_edges) # just add some colours to edges from original image. cv2.imshow('canny demo',dst) lowThreshold = 0 max_lowThreshold = 300 #最大阈值 ratio = 3 kernel_size = 3 #img = cv2.imread('AAA.jpg', cv2.IMREAD_GRAYSCALE) #注释此行图片不灰度化 img = cv2.imread("D:\xiao.jpg") #注释此行上行代码不注释图片灰度化 gray = cv2.imread("D:\xiao.jpg", cv2.IMREAD_GRAYSCALE) cv2.namedWindow('canny demo') cv2.createTrackbar('Min threshold','canny demo',lowThreshold, max_lowThreshold, CannyThreshold) CannyThreshold(0) # initialization if cv2.waitKey(0) == 27: cv2.destroyAllWindows()
抱歉,我的回答有误。这段代码是使用OpenCV库进行Canny边缘检测的操作,并且使用了滑动条来调节最小阈值。其中,首先对输入的图像进行了高斯模糊处理,然后使用Canny函数进行边缘检测,最后使用bitwise_and函数将原图像和检测出的边缘进行合并并显示出来。滑动条的回调函数是CannyThreshold,用于实时更新最小阈值并重新进行边缘检测和显示。如果按下ESC键,则关闭窗口。
相关推荐
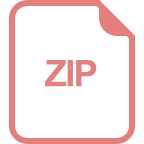
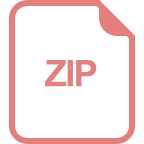












