任意选择核函数和参数实现以上实验
时间: 2024-09-12 11:13:39 浏览: 44
由于您没有提供具体的实验环境或上下文,我将假设您是在询问如何在Python中使用支持向量机(SVM)算法,并通过选择不同的核函数和参数来实现分类或回归任务。在Python中,我们通常使用`scikit-learn`这个机器学习库来实现这些功能。
以下是一个使用`scikit-learn`库中`SVC`(支持向量分类器)的示例代码,它展示了如何选择不同的核函数和参数进行分类:
```python
from sklearn import svm
from sklearn.datasets import load_iris
from sklearn.model_selection import train_test_split
from sklearn.metrics import classification_report
# 加载数据集,这里以鸢尾花数据集为例
data = load_iris()
X, y = data.data, data.target
# 划分训练集和测试集
X_train, X_test, y_train, y_test = train_test_split(X, y, test_size=0.3, random_state=42)
# 创建支持向量分类器实例,这里使用RBF核函数
clf_rbf = svm.SVC(kernel='rbf', gamma='scale')
# 训练模型
clf_rbf.fit(X_train, y_train)
# 预测测试集
predictions_rbf = clf_rbf.predict(X_test)
# 打印分类报告
print(classification_report(y_test, predictions_rbf))
# 如果您想尝试其他核函数,比如多项式核,可以如下操作:
clf_poly = svm.SVC(kernel='poly', degree=3, gamma='scale')
clf_poly.fit(X_train, y_train)
predictions_poly = clf_poly.predict(X_test)
print(classification_report(y_test, predictions_poly))
# 或者线性核函数:
clf_linear = svm.SVC(kernel='linear')
clf_linear.fit(X_train, y_train)
predictions_linear = clf_linear.predict(X_test)
print(classification_report(y_test, predictions_linear))
```
在这个例子中,我们首先加载了一个内置的鸢尾花数据集,并将其分为训练集和测试集。然后,我们创建了三个不同的`SVC`分类器实例,分别使用了不同的核函数:径向基函数(RBF)、多项式核和线性核。我们还展示了如何设置核函数的参数,例如`degree`用于多项式核,以及如何训练和评估模型。
请注意,`gamma`参数控制了数据映射到新特征空间后的分布,这里使用了默认值`'scale'`,它会根据数据的维度自动选择。
阅读全文
相关推荐
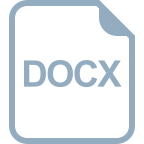
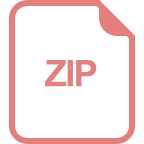
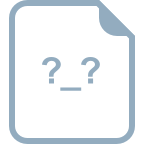
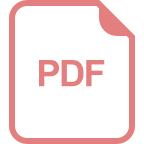
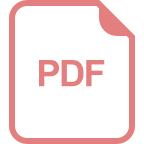
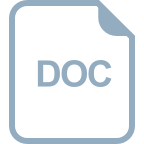
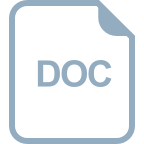
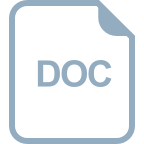
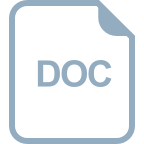
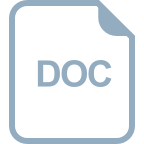
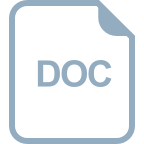
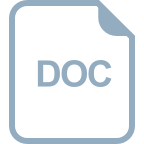
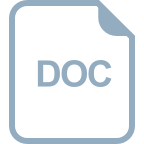
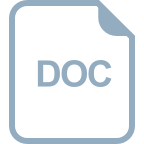
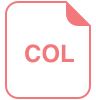
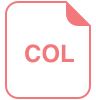
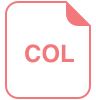
